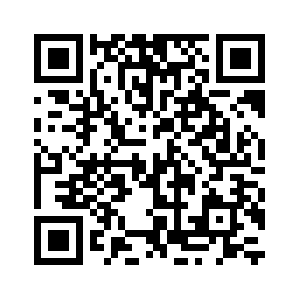
AI-Driven Smart Auditory Health Systems: Bridging Audiology and Public Health in Low- and Middle-Income Countries
Public Health受け取った 11 Nov 2024 受け入れられた 04 Dec 2024 オンラインで公開された 05 Dec 2024
Focusing on Biology, Medicine and Engineering ISSN: 2995-8067 | Quick Google Scholar
Next Full Text
Will SpaceX Send Humans to Mars in 2028?
受け取った 11 Nov 2024 受け入れられた 04 Dec 2024 オンラインで公開された 05 Dec 2024
Hearing loss is a critical global health issue that affects over 1.5 billion people worldwide, with a disproportionate burden in Low- and Middle-Income Countries (LMICs). These regions face significant challenges, including limited access to audiological services, a shortage of healthcare professionals, and a lack of affordable hearing solutions. These barriers lead to delayed diagnoses, inadequate management, and a significant negative impact on individuals' quality of life, education, and employment opportunities. The advent of Artificial Intelligence (AI) and advanced technologies offers innovative pathways to address these longstanding challenges. This review introduces the AI-driven smart Auditory Health Systems (SAHS) concept. This holistic approach integrates AI, wearable devices, Internet of Things (IoT) technology, and big data analytics to enhance the prevention, diagnosis, and management of auditory disorders. SAHS systems can provide real-time monitoring, early detection of hearing loss, and personalized care solutions tailored to individual and population needs. These systems offer community-level interventions, including noise pollution monitoring and data-driven public health strategies. Focusing on the LMIC context, this review explores the technological framework, applications, ethical considerations, and logistical challenges of implementing SAHS. By leveraging these advanced technologies, SAHS has the potential to bridge gaps in healthcare access, improve health outcomes, and transform auditory healthcare delivery in resource-constrained settings. This review underscores the importance of collaborative efforts in research, policy development, and capacity building to ensure equitable adoption of SAHS, thereby addressing hearing health disparities globally.
Hearing loss is a pervasive global health issue, with the World Health Organization (WHO) estimating that over 1.5 billion people live with some degree of hearing loss, and approximately 430 million require rehabilitation services [
, ]. The burden is particularly pronounced in low- and middle-income countries (LMICs), where 80% of individuals with hearing loss reside [ ]. In these regions, access to audiological services is often limited due to factors such as insufficient healthcare infrastructure, a shortage of trained professionals, and financial constraints [ ]. Consequently, many individuals do not receive timely diagnoses or appropriate interventions, leading to adverse outcomes in communication, education, employment, and overall quality of life [ ]. Traditional approaches to hearing healthcare in LMICs have been challenged by these systemic barriers. Conventional hearing aids and cochlear implants, while effective, are often inaccessible due to high costs and the need for specialized fitting and maintenance. Moreover, public health initiatives targeting hearing loss prevention and management are frequently underfunded and lack integration into broader healthcare strategies [ ]. This situation underscores the need for innovative solutions that can overcome these obstacles and provide scalable, cost-effective auditory healthcare [ ]. The rapid advancement of Artificial Intelligence (AI) and digital health technologies presents a transformative opportunity to address these challenges. AI has demonstrated significant potential in various healthcare domains, including diagnostics, personalized medicine, and predictive analytics [ , ]. In audiology, AI can enhance the functionality of hearing devices, facilitate early detection of hearing impairments, and support data-driven public health interventions [ ]. When integrated with wearable technology and the Internet of Things (IoT), AI enables continuous monitoring of auditory health, providing real-time feedback and personalized care [ ].This review paper introduces the concept of AI-driven smart Auditory Health Systems (SAHS), which leverage AI, wearable devices, IoT, and big data analytics to create a comprehensive framework for auditory healthcare [
]. SAHS aims to bridge the gap between individual patient care and public health strategies, offering solutions that are particularly relevant to the LMIC context. By utilizing readily available technologies and scalable platforms, SAHS has the potential to overcome existing barriers and improve access to quality auditory healthcare in resource-constrained settings.In the following sections, we will explore the technological framework underpinning SAHS, discuss their applications at individual and population levels, examine ethical and logistical challenges, and consider future directions for research and implementation. Through this comprehensive analysis, we aim to highlight the potential of SAHS to transform auditory healthcare and contribute to global health equity.
The foundation of Smart Auditory Health Systems (SAHS) lies in the integration of advanced technologies that collectively enhance auditory healthcare delivery [
]. In the context of Low- and Middle-Income Countries (LMICs), where healthcare resources are often limited, the adoption of such technologies offers a pathway to overcoming existing barriers and improving access to quality auditory care. Artificial Intelligence (AI) has emerged as a transformative force in healthcare, offering capabilities that extend beyond traditional diagnostic and therapeutic approaches [ ]. In audiology, AI algorithms can analyze complex datasets to identify patterns indicative of hearing impairments, enabling early detection and intervention. For instance, machine learning models can process audiometric data to predict the onset of hearing loss, facilitating proactive management strategies [ , ]. Additionally, AI-driven speech recognition technologies enhance the performance of hearing aids by adapting to diverse acoustic environments, thereby improving user experience and satisfaction.Wearable devices, such as smart hearing aids and noise exposure monitors, play a crucial role in SAHS by providing continuous monitoring of auditory health metrics [
]. The integration of wearable devices with AI-driven health systems has significantly advanced healthcare delivery in recent years, particularly in areas like continuous monitoring and early diagnosis. Studies have shown the potential of wearable sensors to monitor physiological signals accurately, supporting personalized healthcare approaches [ ]. These technologies are particularly relevant in auditory health, where continuous monitoring of noise exposure and hearing conditions can mitigate long-term impairments [ ]. Recent advancements in machine learning have also facilitated the development of integrated health platforms that collect, analyze, and interpret large-scale health data, enabling precise interventions and better resource allocation [ ]. These innovations underscore the transformative potential of AI-powered wearable systems in addressing global health disparities.Smart Auditory Health Systems (SAHS) rely on a variety of sensors and instrumentation for data collection, processing, and actionable feedback. Key components include wearable hearing aids integrated with Digital Signal Processing (DSP) chips, environmental noise sensors, and IoT-enabled monitoring devices. For instance, noise dosimeters equipped with MEMS microphones can measure noise levels with high precision at frequencies ranging from 20 Hz to 20 kHz. These devices often support Bluetooth or Wi-Fi connectivity, enabling real-time data transmission to centralized SAHS platforms [
]. Additionally, personal hearing aids with AI integration are embedded with advanced feedback suppression systems and adaptive beamforming microphones to optimize user experience in diverse acoustic environments [ ].In terms of costs, wearable hearing aids with AI capabilities typically range between $300 and $1,500 per unit, depending on features such as noise cancellation, real-time processing, and connectivity options [
]. IoT-enabled environmental noise sensors, used for community monitoring, cost approximately $100–$500 per device, with recurring expenses for data storage and analysis services estimated at $50–$100 annually per deployment [ ]. These costs highlight the affordability of SAHS components relative to their transformative potential in LMICs. The integration of these devices with the Internet of Things (IoT) enables the collection and transmission of real-time data to centralized platforms for analysis. Effective implementation of Smart Auditory Health Systems (SAHS) depends on monitoring network outputs from wearable devices. These devices transmit data on noise levels, auditory health metrics, and performance through IoT platforms. Real-time monitoring ensures data accuracy, system reliability, and prompt interventions. Centralized dashboards aggregate and visualize data, detecting issues such as device malfunctions or connectivity problems. AI algorithms can identify anomalies, enabling timely maintenance or recalibration. Location-specific monitoring also supports targeted interventions, such as identifying high-noise urban areas for immediate action or allocating resources in rural regions at risk. Robust network systems with fail-safes like data buffering and redundancy protocols prevent data loss and enhance system reliability, ensuring SAHS operates effectively across diverse settings. In LMICs, where access to healthcare facilities may be limited, wearable devices offer a practical solution for remote monitoring and management of hearing health [ ]. For example, IoT-enabled noise sensors can be deployed in urban areas to monitor environmental noise levels, providing data that inform public health interventions aimed at reducing noise pollution [ ].The aggregation of data from wearable devices and IoT sensors facilitates the application of big data analytics, which can uncover population-level trends and inform public health strategies. In LMICs, where epidemiological data on hearing loss may be scarce, big data analytics can provide valuable insights into the prevalence and distribution of auditory health issues [
, ]. This information is essential for designing targeted interventions, allocating resources effectively, and evaluating the impact of public health programs. For instance, analysis of noise exposure data can identify high-risk communities, prompting the implementation of noise reduction policies and awareness campaigns [ ].By leveraging AI, wearable devices, IoT, and big data analytics, SAHS creates a comprehensive ecosystem that addresses both individual and population-level auditory health needs. This technological framework is particularly advantageous in LMICs, where traditional healthcare infrastructure may be inadequate to meet the growing demand for auditory care. The scalability and adaptability of SAHS make them well-suited to the diverse challenges present in these settings, offering a pathway to improved hearing health outcomes and greater health equity.
The methodology of this study was designed to provide a comprehensive review of the potential of AI-driven smart Auditory Health Systems (SAHS) in addressing auditory healthcare challenges, particularly in low- and middle-income countries (LMICs). This approach involved a systematic analysis of existing literature to identify technological frameworks, applications, ethical considerations, and implementation challenges relevant to SAHS in resource-constrained settings. To collect relevant information, a systematic search strategy was employed using prominent electronic databases, including PubMed, Scopus, IEEE Xplore, and Google Scholar. Keywords such as "AI in audiology," "hearing loss technologies," "digital health in LMICs," "smart auditory health systems," and "public health interventions for hearing loss" were utilized to refine the search. Boolean operators (AND/OR) were applied to ensure the inclusion of studies covering intersections of these themes. The search was restricted to peer-reviewed articles published in English between 2013 and 2024 to capture recent advancements in the field. The inclusion criteria for this review were defined to ensure the relevance and quality of selected studies. Articles were included if they explored the role of Artificial Intelligence (AI), wearable devices, Internet of Things (IoT), or big data analytics in auditory healthcare. Studies that focused on hearing loss prevention, diagnosis, or management in LMIC contexts were prioritized. Additionally, research addressing ethical considerations and implementation challenges of digital health systems was included. Studies unrelated to auditory health, those focusing solely on high-income countries, or articles lacking empirical evidence were excluded from the review to maintain focus and rigor. Data were extracted from the selected articles using a standardized format to gather key information. This included the objectives of the study, the technologies employed (such as AI, IoT, or wearables), the target populations and settings, and the results and conclusions regarding the effectiveness of SAHS. Extracted data were synthesized thematically to identify recurring trends and critical gaps. Key themes were organized into technological frameworks, applications, ethical considerations, and areas for future research.
Environmental noise is a significant public health concern, particularly in urban areas of LMICs where industrial activity, traffic congestion, and unregulated urbanization contribute to excessive noise exposure. Chronic exposure to high noise levels, often exceeding the recommended 85 dB(A) threshold, is linked to irreversible hearing damage, increased stress levels, and cardiovascular health risks [
]. AI-assisted SAHS can play a pivotal role in mitigating the impact of environmental noise by deploying IoT-enabled noise sensors for real-time monitoring and hotspot identification. For instance, wearable noise dosimeters integrated into SAHS platforms allow for individual-level tracking of sound exposure, alerting users when thresholds are exceeded. Managing environmental noise effectively requires a multi-stakeholder approach, including policymakers, urban planners, and healthcare providers. Investments in noise monitoring infrastructure, coupled with stringent enforcement of noise control laws, are essential to reducing noise-induced hearing loss and improving overall public health outcomes.The implementation of Smart Auditory Health Systems (SAHS) in Low- and Middle-Income Countries (LMICs) offers a multifaceted approach to addressing auditory health challenges at both individual and community levels. By leveraging advanced technologies, SAHS can enhance the prevention, diagnosis, and management of hearing loss, thereby improving overall public health outcomes.
In LMICs, access to audiological services is often limited due to a shortage of healthcare professionals and financial constraints. SAHS can mitigate these challenges by providing personalized auditory health solutions through wearable devices integrated with Artificial Intelligence (AI). For instance, AI-powered hearing aids can automatically adjust settings based on the user's environment, improving speech recognition and overall hearing experience [
, ]. Additionally, wearable noise exposure monitors can alert individuals to hazardous sound levels, enabling proactive measures to prevent noise-induced hearing loss. These technologies empower individuals to take control of their hearing health, reducing reliance on scarce healthcare resources.At the community level, SAHS facilitates public health strategies aimed at preventing and managing hearing loss. The integration of Internet of Things (IoT) devices, such as environmental noise sensors, allows for real-time monitoring of noise pollution in urban areas [
]. Data collected from these sensors can inform policymakers about noise pollution hotspots, leading to targeted interventions such as traffic management and urban planning to reduce noise exposure [ ]. Furthermore, community health workers equipped with mobile applications connected to SAHS can conduct hearing screenings and disseminate educational materials, raising awareness about hearing health and promoting preventive behaviors.Occupational noise-induced hearing loss is a significant concern in LMICs, particularly in industries such as manufacturing and construction. SAHS can monitor workplace noise levels and provide feedback to both employers and employees, facilitating the implementation of effective hearing conservation programs [
, ]. In educational settings, SAHS can support school-based hearing screening programs, enabling early detection of hearing impairments among children. Early intervention is crucial for academic success and social development, and SAHS provides the tools necessary for the timely identification and management of hearing issues in school-aged populations.While the potential benefits of Smart Auditory Health Systems (SAHS) in Low- and Middle-Income Countries (LMICs) are substantial, their implementation is accompanied by several ethical and logistical challenges that must be carefully addressed. The collection and processing of sensitive auditory health data through SAHS raise significant privacy concerns. In LMICs, where data protection regulations may be less stringent or inconsistently enforced, the risk of unauthorized access or misuse of personal health information is heightened. Ensuring robust data encryption, establishing clear consent protocols, and adhering to international data protection standards are essential to maintaining public trust and protecting individual privacy [
].The digital divide presents a significant barrier to the widespread adoption of SAHS in LMICs. Training users and their family members is critical for the effective use of Smart Auditory Health Systems (SAHS). This includes guidance on operating wearable devices, adjusting settings, and interpreting alerts. Training can be provided through community workshops, instructional videos, and mobile apps tailored to local languages. Hands-on sessions led by healthcare workers allow users and families to practice handling and troubleshooting devices. Empowering families is especially important in LMICs, where healthcare access is limited. Trained family members can assist with monitoring device performance and ensuring consistent use, particularly for children or elderly users. Accessible and culturally appropriate training materials enhance user engagement and the overall success of SAHS. Many individuals lack access to the necessary technology, such as smartphones or internet connectivity, required to utilize SAHS effectively [
]. Additionally, the cost of wearable devices may be prohibitive for low-income populations. Addressing these disparities requires targeted interventions, such as subsidized device programs, community-based digital literacy initiatives, and the development of low-cost, user-friendly technologies tailored to the local context.The effectiveness of SAHS depends on the seamless integration of various devices and platforms. However, the lack of standardized protocols and interoperability between different technologies can hinder data sharing and system efficiency. Developing universal standards and fostering collaboration among technology developers, healthcare providers, and policymakers are crucial steps toward creating cohesive and functional SAHS ecosystems.
Implementing SAHS in LMICs necessitates building local capacity in AI and digital health. Training healthcare professionals, technicians, and community health workers to operate and maintain these systems is essential for sustainability. Moreover, involving local stakeholders in the design and deployment of SAHS ensures that the systems are culturally appropriate and aligned with the specific needs of the community.
The introduction of Smart Auditory Health Systems (SAHS) in Low- and Middle-Income Countries (LMICs) holds the potential to revolutionize auditory healthcare by addressing deep-rooted disparities and aligning with broader public health priorities. Hearing loss remains a significant yet underappreciated health concern in LMICs, where resource limitations and systemic challenges exacerbate the problem. SAHS, through the integration of Artificial Intelligence (AI), wearable devices, the Internet of Things (IoT), and big data analytics, offers innovative solutions that are both scalable and impactful [
, ]. These systems promise to bridge gaps in access, affordability, and efficiency, ensuring more equitable auditory healthcare delivery. One of the most notable advantages of SAHS is its ability to provide real-time monitoring and actionable, data-driven insights [ ]. This capability is particularly valuable in rural and underserved areas, where traditional audiological care is often unavailable or inadequate. For instance, wearable devices equipped with noise exposure monitors can serve as early-warning systems for individuals working in high-risk environments, such as industrial and construction sites [ , ]. These devices not only alert users to harmful noise levels but also enable proactive interventions to prevent irreversible hearing loss. This represents a shift from reactive to preventive healthcare, which is critical for addressing the burden of auditory disorders in LMICs. AI-driven hearing aids further enhance the individual-level benefits of SAHS. AI-assisted devices offer distinct advantages over non-AI-assisted systems, primarily in personalization and proactive care. While traditional auditory devices rely on static settings, AI-powered systems dynamically adapt to the user's environment, enhancing speech recognition and noise filtering for improved user satisfaction [ ]. Additionally, AI-driven wearable devices enable real-time monitoring and predictive interventions, such as alerts for harmful noise exposure, which are particularly beneficial in high-risk occupational settings. These capabilities underscore the transformative potential of AI in auditory healthcare, enabling a shift from reactive to preventive solutions and ensuring better health outcomes. Unlike conventional hearing aids, which often provide generic amplification, AI-powered devices use advanced algorithms to adapt to the unique needs of each user. These devices can analyze environmental sounds in real-time, optimize settings for improved speech recognition, and provide a more personalized auditory experience [ ]. Such innovations significantly improve user satisfaction and long-term adherence, addressing one of the key challenges associated with hearing aid use in LMICs. However, the successful implementation of SAHS in LMICs is not without challenges. The high cost of wearable devices remains a significant barrier to adoption, particularly in low-income communities [ , ]. The predictive power of AI-driven SAHS models is critically dependent on the quality and diversity of retrospective data used for training. Retrospective datasets often contain inherent biases, such as overrepresentation of certain populations or underreporting of noise exposure levels in rural areas. Despite these limitations, machine learning approaches in audiology have demonstrated predictive accuracies ranging from 85% to 92% in identifying high-risk groups for hearing loss when trained on diverse datasets [ ]. In LMICs, where retrospective data may be incomplete or skewed, integrating real-time data from wearable IoT devices significantly enhances predictive accuracy by providing contextualized and population-specific insights. These advancements underscore the need to invest in data collection infrastructure and harmonized protocols to optimize predictive outcomes globally in SAHS implementations. Many families in LMICs cannot afford advanced hearing aids or noise monitors, creating a stark divide in access to these life-enhancing technologies. Innovative financing models, such as microloans, device leasing, or subsidies, could play a pivotal role in making SAHS more accessible. Public-private partnerships could also facilitate the distribution of affordable devices by leveraging the resources and expertise of both sectors. Governments and international organizations must prioritize the inclusion of auditory health technologies in public health programs to ensure that the benefits of SAHS reach the most vulnerable populations [ ]. Another critical consideration is the integration of SAHS into existing healthcare frameworks. LMICs often rely heavily on primary care networks and community health worker programs to deliver basic healthcare services. Embedding SAHS into these structures could enhance their reach and impact without overburdening already stretched healthcare systems. For example, community health workers equipped with mobile devices connected to SAHS platforms could perform hearing screenings, distribute wearable devices, and provide education on hearing health. Such an approach would not only improve early detection and intervention rates but also strengthen the overall capacity of healthcare systems in these regions.Beyond individual care, the population-level benefits of SAHS hold immense potential to drive systemic change. By aggregating data from wearable devices and IoT sensors, SAHS can generate insights into community-wide trends, such as noise pollution levels and hearing loss prevalence. This information is invaluable for policymakers, enabling evidence-based urban planning, occupational health regulations, and public education campaigns. For instance, data on noise pollution hotspots could inform zoning laws and infrastructure development, while insights into occupational noise exposure could lead to stricter workplace safety standards. Public health campaigns leveraging these insights could also raise awareness about the importance of hearing protection and preventive care, fostering a culture of proactive auditory health. However, realizing the full potential of SAHS requires substantial investments in digital health infrastructure and regulatory frameworks. LMICs must address critical gaps in internet connectivity, data storage, and cybersecurity to support the deployment of these advanced systems. Equally important is the establishment of ethical guidelines to govern the use of SAHS technologies. Issues such as data privacy, algorithmic bias, and equitable access must be carefully managed to prevent unintended consequences and ensure that these technologies benefit all segments of the population.
While this review highlights the transformative potential of Smart Auditory Health Systems (SAHS) in Low- and Middle-Income Countries (LMICs), it is not without limitations. The primary limitation is the lack of large-scale empirical data to validate the long-term effectiveness of SAHS across diverse settings. Retrospective datasets used in training AI models may introduce biases, impacting the generalizability of results. Additionally, the high cost of devices and limited digital infrastructure in LMICs pose significant barriers to widespread adoption. Ethical concerns, particularly regarding data privacy and accessibility for vulnerable populations, remain unresolved and warrant further investigation.
Future research should focus on conducting pilot studies to assess the real-world effectiveness of SAHS in improving auditory health outcomes. Efforts should also prioritize developing low-cost, durable devices tailored to resource-constrained environments. Moreover, collaboration with local stakeholders is crucial to designing culturally appropriate and scalable training programs. Expanding the integration of SAHS with existing healthcare frameworks and exploring public-private partnerships can also facilitate broader implementation. Addressing these limitations through targeted research and policy initiatives will be pivotal in realizing the full potential of SAHS to bridge auditory health disparities globally.
Smart Auditory Health Systems (SAHS) leverage AI, IoT, and big data analytics to address critical challenges in auditory healthcare, particularly in LMICs. Key findings highlight SAHS' potential for real-time monitoring, early detection, and tailored interventions. However, barriers like device costs, digital infrastructure, and training limitations must be addressed. Recommendations include prioritizing low-cost devices, expanding digital health frameworks, and fostering public-private partnerships. The take-home message is clear: SAHS offers a scalable and transformative solution for auditory health equity, but collaborative efforts in research, policy, and implementation are essential to bridge existing gaps and empower underserved communities globally.
Issue: AI-generated content was found to be 97% (Quillbot).
Author correspondence: On intimating the author of the AI content found and the need for attributing/declaring the use of AI tools, the author confirmed to have not used any tools for AI-generation of content and only used Grammarly for language correction.
Inference: As AI detectors are not absolute, the author's confirmation of not using any AI tools for content generation was accepted, thus, attribution was unnecessary.
Schuh M, Bush ML. Defining disparities in cochlear implantation through the social determinants of health. Semin Hear [Internet]. 2021 Nov [cited 2024 Dec 4];42(04):321-30. Available from: http://www.thieme-connect.de/DOI/DOI?10.1055/s-0041-1739282
Chadha S, Kamenov K, Cieza A. The world report on hearing, 2021. Bull World Health Organ [Internet]. 2021 Apr 1 [cited 2024 Dec 4];99(4):242-242A. Available from: https://www.ncbi.nlm.nih.gov/pmc/articles/PMC8085630/pdf/BLT.21.285643.pdf/
Adeyemo A, Ogunkeyede S, Dania O. Hearing healthcare gaps in LMICS: snapshot from a semi-urban community in Nigeria. Afr Health Sci. 2021 Jun;21(2):912-918. doi: 10.4314/ahs.v21i2.53. PMID: 34795751; PMCID: PMC8568223.
Joubert K. Community-based audiology services: An effective strategy for the prevention of hearing loss in rural communities. In: Khoza-Shangase K, editor. Preventive Audiology: An African perspective [Internet]. Cape Town: AOSIS; 2022. Chapter 4. PMID: 38446933.
Sharkiya SH. Quality communication can improve patient-centred health outcomes among older patients: a rapid review. BMC Health Serv Res [Internet]. 2023 Aug 22 [cited 2024 Dec 4];23(1):886. Available from: https://bmchealthservres.biomedcentral.com/articles/10.1186/s12913-023-09869-8
Pratt SR. Profound Hearing Loss: Addressing Barriers to Hearing Healthcare. Semin Hear. 2018 Nov;39(4):428-436. doi: 10.1055/s-0038-1670708. Epub 2018 Oct 26. PMID: 30374213; PMCID: PMC6203460.
Reavis KM, Tremblay KL, Saunders G. How Can Public Health Approaches and Perspectives Advance Hearing Health Care? Ear Hear. 2016 Jul-Aug;37(4):376-80. doi: 10.1097/AUD.0000000000000321. PMID: 27232072; PMCID: PMC5024732.
Wubineh BZ, Deriba FG, Woldeyohannis MM. Exploring the opportunities and challenges of implementing artificial intelligence in healthcare: A systematic literature review. Urol Oncol. 2024 Mar;42(3):48-56. doi: 10.1016/j.urolonc.2023.11.019. Epub 2023 Dec 14. PMID: 38101991.
Alowais SA, Alghamdi SS, Alsuhebany N, Alqahtani T, Alshaya AI, Almohareb SN, Aldairem A, Alrashed M, Bin Saleh K, Badreldin HA, Al Yami MS, Al Harbi S, Albekairy AM. Revolutionizing healthcare: the role of artificial intelligence in clinical practice. BMC Med Educ. 2023 Sep 22;23(1):689. doi: 10.1186/s12909-023-04698-z. PMID: 37740191; PMCID: PMC10517477.
AlSamhori JF, AlSamhori ARF, Amourah RM, AlQadi Y, Koro ZW, Haddad TRA, et al. Artificial intelligence for hearing loss prevention, diagnosis, and management. J Med Surg Public Health [Internet]. 2024 Aug [cited 2024 Nov 26];3:100133. Available from: https://linkinghub.elsevier.com/retrieve/pii/S2949916X24000860
Wang WH, Hsu WS. Integrating Artificial Intelligence and Wearable IoT System in Long-Term Care Environments. Sensors (Basel). 2023 Jun 26;23(13):5913. doi: 10.3390/s23135913. PMID: 37447763; PMCID: PMC10346723.
Shajari S, Kuruvinashetti K, Komeili A, Sundararaj U. The Emergence of AI-Based Wearable Sensors for Digital Health Technology: A Review. Sensors (Basel). 2023 Nov 29;23(23):9498. doi: 10.3390/s23239498. PMID: 38067871; PMCID: PMC10708748.
Swanepoel W. eHealth Technologies Enable more Accessible Hearing Care. Semin Hear. 2020 May;41(2):133-140. doi: 10.1055/s-0040-1708510. Epub 2020 Apr 7. PMID: 32269417; PMCID: PMC7138638.
Olawade DB, Wada OZ, Odetayo A, David-Olawade AC, Asaolu F, Eberhardt J. Enhancing mental health with artificial intelligence: Current trends and future prospects. J Med Surg Public Health [Internet]. 2024 Aug [cited 2024 Aug 10];3:100099. Available from: https://linkinghub.elsevier.com/retrieve/pii/S2949916X24000525
Zhao Y, Li J, Zhang M, Lu Y, Xie H, Tian Y, Qiu W. Machine Learning Models for the Hearing Impairment Prediction in Workers Exposed to Complex Industrial Noise: A Pilot Study. Ear Hear. 2019 May/Jun;40(3):690-699. doi: 10.1097/AUD.0000000000000649. PMID: 30142102; PMCID: PMC6493679.
Wang Y, Yao X, Wang D, Ye C, Xu L. A machine learning screening model for identifying the risk of high-frequency hearing impairment in a general population. BMC Public Health. 2024 Apr 25;24(1):1160. doi: 10.1186/s12889-024-18636-1. PMID: 38664666; PMCID: PMC11044481.
Jafleh EA, Alnaqbi FA, Almaeeni HA, Faqeeh S, Alzaabi MA, Al Zaman K. The Role of Wearable Devices in Chronic Disease Monitoring and Patient Care: A Comprehensive Review. Cureus. 2024 Sep 8;16(9):e68921. doi: 10.7759/cureus.68921. PMID: 39381470; PMCID: PMC11461032.
Pigini L, Bovi G, Panzarino C, Gower V, Ferratini M, Andreoni G, Sassi R, Rivolta MW, Ferrarin M. Pilot Test of a New Personal Health System Integrating Environmental and Wearable Sensors for Telemonitoring and Care of Elderly People at Home (SMARTA Project). Gerontology. 2017;63(3):281-286. doi: 10.1159/000455168. Epub 2017 Jan 19. PMID: 28099965.
Baig MM, Afifi S, GholamHosseini H, Mirza F. A Systematic Review of Wearable Sensors and IoT-Based Monitoring Applications for Older Adults - a Focus on Ageing Population and Independent Living. J Med Syst. 2019 Jun 15;43(8):233. doi: 10.1007/s10916-019-1365-7. PMID: 31203472.
Pan H, Lin Z, Fu P, Qi Y, Wang W. Modeling intra and inter-modality incongruity for multi-modal sarcasm detection. In: Findings of the Association for Computational Linguistics: EMNLP 2020 [Internet]. Online: Association for Computational Linguistics; 2020 [cited 2024 Dec 4]. p. 1383-92. Available from: https://www.aclweb.org/anthology/2020.findings-emnlp.124
Salamone F, Masullo M, Sibilio S. Wearable Devices for Environmental Monitoring in the Built Environment: A Systematic Review. Sensors (Basel). 2021 Jul 10;21(14):4727. doi: 10.3390/s21144727. PMID: 34300467; PMCID: PMC8309593.
Hearing Aid UK [Internet]. [cited 2024 Nov 26]. Can AI help your hearing? Available from: https://www.hearingaid.org.uk/hearing-aid-technology/how-artificial-intelligence-can-help-your-hearing
Saldana-Barrios JJ, Aguilar E, Ng W, Orocu R. Designing an IoT-Based System for Monitoring Noise Levels in the Computer Science Faculty and Library of the Technological University of Panama. Sensors (Basel). 2023 Nov 10;23(22):9083. doi: 10.3390/s23229083. PMID: 38005471; PMCID: PMC10675386.
Casillo M, Cecere L, Colace F, Lorusso A, Santaniello D. Integrating the Internet of Things (IoT) in SPA medicine: Innovations and challenges in digital wellness. Computers [Internet]. 2024 Mar 6 [cited 2024 Nov 26];13(3):67. Available from: https://www.mdpi.com/2073-431X/13/3/67
Ramadan MNA, Ali MAH, Khoo SY, Alkhedher M, Alherbawi M. Real-time IoT-powered AI system for monitoring and forecasting of air pollution in industrial environment. Ecotoxicol Environ Saf. 2024 Sep 15;283:116856. doi: 10.1016/j.ecoenv.2024.116856. Epub 2024 Aug 15. PMID: 39151373.
Wasmann JA, Lanting CP, Huinck WJ, Mylanus EAM, van der Laak JWM, Govaerts PJ, Swanepoel W, Moore DR, Barbour DL. Computational Audiology: New Approaches to Advance Hearing Health Care in the Digital Age. Ear Hear. 2021 Nov-Dec 01;42(6):1499-1507. doi: 10.1097/AUD.0000000000001041. PMID: 33675587; PMCID: PMC8417156.
Ganek HV, Madubueze A, Merritt CE, Bhutta ZA. Prevalence of hearing loss in children living in low- and middle-income countries over the last 10 years: A systematic review. Dev Med Child Neurol. 2023 May;65(5):600-610. doi: 10.1111/dmcn.15460. Epub 2022 Nov 17. PMID: 36397253.
Moroe NF. Early detection and management of occupational and environmental noise. In: Khoza-Shangase K, editor. Preventive audiology: An African perspective [Internet]. Cape Town: AOSIS; 2022 [cited 2024 Nov 26]. Available from: http://www.ncbi.nlm.nih.gov/books/NBK601304/
Patel H, Davy P, Tollemache C, Talbot N, Salmond J, Williams DE. Evaluating the efficacy of targeted traffic management interventions: A novel methodology for determining the composition of particulate matter in urban air pollution hotspots. Sci Total Environ. 2024 Nov 15;951:175414. doi: 10.1016/j.scitotenv.2024.175414. Epub 2024 Aug 8. PMID: 39127221.
Fabry DA, Bhowmik AK. Improving Speech Understanding and Monitoring Health with Hearing Aids Using Artificial Intelligence and Embedded Sensors. Semin Hear. 2021 Aug;42(3):295-308. doi: 10.1055/s-0041-1735136. Epub 2021 Sep 24. PMID: 34594091; PMCID: PMC8463124.
Lie A, Skogstad M, Johannessen HA, Tynes T, Mehlum IS, Nordby KC, Engdahl B, Tambs K. Occupational noise exposure and hearing: a systematic review. Int Arch Occup Environ Health. 2016 Apr;89(3):351-72. doi: 10.1007/s00420-015-1083-5. Epub 2015 Aug 7. PMID: 26249711; PMCID: PMC4786595.
Chen KH, Su SB, Chen KT. An overview of occupational noise-induced hearing loss among workers: epidemiology, pathogenesis, and preventive measures. Environ Health Prev Med. 2020 Oct 31;25(1):65. doi: 10.1186/s12199-020-00906-0. PMID: 33129267; PMCID: PMC7603754.
Hussein R, Griffin AC, Pichon A, Oldenburg J. A guiding framework for creating a comprehensive strategy for mHealth data sharing, privacy, and governance in low- and middle-income countries (LMICs). J Am Med Inform Assoc. 2023 Mar 16;30(4):787-794. doi: 10.1093/jamia/ocac198. PMID: 36259962; PMCID: PMC10018261.
Saeed SA, Masters RM. Disparities in Health Care and the Digital Divide. Curr Psychiatry Rep. 2021 Jul 23;23(9):61. doi: 10.1007/s11920-021-01274-4. PMID: 34297202; PMCID: PMC8300069.
Rejeb A, Rejeb K, Treiblmaier H, Appolloni A, Alghamdi S, Alhasawi Y, et al. The Internet of Things (IoT) in healthcare: Taking stock and moving forward. Internet of Things [Internet]. 2023 Jul 1;22:100721. Available from: https://www.sciencedirect.com/science/article/pii/S2542660523000446
Alahi MEE, Sukkuea A, Tina FW, Nag A, Kurdthongmee W, Suwannarat K, Mukhopadhyay SC. Integration of IoT-Enabled Technologies and Artificial Intelligence (AI) for Smart City Scenario: Recent Advancements and Future Trends. Sensors (Basel). 2023 May 30;23(11):5206. doi: 10.3390/s23115206. PMID: 37299934; PMCID: PMC10256108.
Valentin G, Nielsen CV, Nielsen AM, Tonnesen M, Bliksted KL, Jensen KT, Ingerslev K, Maribo T, Oestergaard LG. Bridging Inequity Gaps in Healthcare Systems While Educating Future Healthcare Professionals-The Social Health Bridge-Building Programme. Int J Environ Res Public Health. 2023 Sep 27;20(19):6837. doi: 10.3390/ijerph20196837. PMID: 37835107; PMCID: PMC10572531.
Lu L, Zhang J, Xie Y, Gao F, Xu S, Wu X, Ye Z. Wearable Health Devices in Health Care: Narrative Systematic Review. JMIR Mhealth Uhealth. 2020 Nov 9;8(11):e18907. doi: 10.2196/18907. PMID: 33164904; PMCID: PMC7683248.
FasterCapital [Internet]. [cited 2024 Dec 4]. The role of artificial intelligence in hearing solutions. Available from: https://fastercapital.com/keyword/the-role-of-artificial-intelligence-in-hearing-solutions.html
Clark H, Coll-Seck AM, Banerjee A, Peterson S, Dalglish SL, Ameratunga S, et al. A future for the world’s children? A WHO–UNICEF–Lancet Commission. Lancet [Internet]. 2020 Feb 22 [cited 2024 Nov 25];395(10224):605-58. Available from: https://doi.org/10.1016/S0140-6736(19)32540-1
Aslam B, Asghar R, Muzammil S, Shafique M, Siddique AB, Khurshid M, Ijaz M, Rasool MH, Chaudhry TH, Aamir A, Baloch Z. AMR and Sustainable Development Goals: at a crossroads. Global Health. 2024 Oct 17;20(1):73. doi: 10.1186/s12992-024-01046-8. PMID: 39415207; PMCID: PMC11484313
Dillard LK, Der CM, Laplante-Lévesque A, Swanepoel W, Thorne PR, McPherson B, de Andrade V, Newall J, Ramos HD, Kaspar A, Nieman CL, Clark JL, Chadha S. Service delivery approaches related to hearing aids in low- and middle-income countries or resource-limited settings: A systematic scoping review. PLOS Glob Public Health. 2024 Jan 24;4(1):e0002823. doi: 10.1371/journal.pgph.0002823. PMID: 38266001; PMCID: PMC10807760.
Ferdous A, Nishan MDNH, Jahan F. AI-Driven Smart Auditory Health Systems: Bridging Audiology and Public Health in Low- and Middle-Income Countries. IgMin Res. December 05, 2024; 2(12): 950-957. IgMin ID: igmin272; DOI:10.61927/igmin272; Available at: igmin.link/p272
次のリンクを共有した人は、このコンテンツを読むことができます:
1Department of Audiology and Speech-Language Pathology, Bangladesh University of Professionals, Dhaka-1216, Bangladesh
2Department of Public Health, North South University, Dhaka-1229, Bangladesh
Address Correspondence:
MD Nahid Hassan Nishan, MBBS, MPH, Department of Public Health, School of Health and Life Sciences, North South University, Dhaka-1229, Bangladesh, Email: [email protected]
How to cite this article:
Ferdous A, Nishan MDNH, Jahan F. AI-Driven Smart Auditory Health Systems: Bridging Audiology and Public Health in Low- and Middle-Income Countries. IgMin Res. December 05, 2024; 2(12): 950-957. IgMin ID: igmin272; DOI:10.61927/igmin272; Available at: igmin.link/p272
Copyright: © 2024 Ferdous A, et al. This is an open access article distributed under the Creative Commons Attribution License, which permits unrestricted use, distribution, and reproduction in any medium, provided the original work is properly cited.
Schuh M, Bush ML. Defining disparities in cochlear implantation through the social determinants of health. Semin Hear [Internet]. 2021 Nov [cited 2024 Dec 4];42(04):321-30. Available from: http://www.thieme-connect.de/DOI/DOI?10.1055/s-0041-1739282
Chadha S, Kamenov K, Cieza A. The world report on hearing, 2021. Bull World Health Organ [Internet]. 2021 Apr 1 [cited 2024 Dec 4];99(4):242-242A. Available from: https://www.ncbi.nlm.nih.gov/pmc/articles/PMC8085630/pdf/BLT.21.285643.pdf/
Adeyemo A, Ogunkeyede S, Dania O. Hearing healthcare gaps in LMICS: snapshot from a semi-urban community in Nigeria. Afr Health Sci. 2021 Jun;21(2):912-918. doi: 10.4314/ahs.v21i2.53. PMID: 34795751; PMCID: PMC8568223.
Joubert K. Community-based audiology services: An effective strategy for the prevention of hearing loss in rural communities. In: Khoza-Shangase K, editor. Preventive Audiology: An African perspective [Internet]. Cape Town: AOSIS; 2022. Chapter 4. PMID: 38446933.
Sharkiya SH. Quality communication can improve patient-centred health outcomes among older patients: a rapid review. BMC Health Serv Res [Internet]. 2023 Aug 22 [cited 2024 Dec 4];23(1):886. Available from: https://bmchealthservres.biomedcentral.com/articles/10.1186/s12913-023-09869-8
Pratt SR. Profound Hearing Loss: Addressing Barriers to Hearing Healthcare. Semin Hear. 2018 Nov;39(4):428-436. doi: 10.1055/s-0038-1670708. Epub 2018 Oct 26. PMID: 30374213; PMCID: PMC6203460.
Reavis KM, Tremblay KL, Saunders G. How Can Public Health Approaches and Perspectives Advance Hearing Health Care? Ear Hear. 2016 Jul-Aug;37(4):376-80. doi: 10.1097/AUD.0000000000000321. PMID: 27232072; PMCID: PMC5024732.
Wubineh BZ, Deriba FG, Woldeyohannis MM. Exploring the opportunities and challenges of implementing artificial intelligence in healthcare: A systematic literature review. Urol Oncol. 2024 Mar;42(3):48-56. doi: 10.1016/j.urolonc.2023.11.019. Epub 2023 Dec 14. PMID: 38101991.
Alowais SA, Alghamdi SS, Alsuhebany N, Alqahtani T, Alshaya AI, Almohareb SN, Aldairem A, Alrashed M, Bin Saleh K, Badreldin HA, Al Yami MS, Al Harbi S, Albekairy AM. Revolutionizing healthcare: the role of artificial intelligence in clinical practice. BMC Med Educ. 2023 Sep 22;23(1):689. doi: 10.1186/s12909-023-04698-z. PMID: 37740191; PMCID: PMC10517477.
AlSamhori JF, AlSamhori ARF, Amourah RM, AlQadi Y, Koro ZW, Haddad TRA, et al. Artificial intelligence for hearing loss prevention, diagnosis, and management. J Med Surg Public Health [Internet]. 2024 Aug [cited 2024 Nov 26];3:100133. Available from: https://linkinghub.elsevier.com/retrieve/pii/S2949916X24000860
Wang WH, Hsu WS. Integrating Artificial Intelligence and Wearable IoT System in Long-Term Care Environments. Sensors (Basel). 2023 Jun 26;23(13):5913. doi: 10.3390/s23135913. PMID: 37447763; PMCID: PMC10346723.
Shajari S, Kuruvinashetti K, Komeili A, Sundararaj U. The Emergence of AI-Based Wearable Sensors for Digital Health Technology: A Review. Sensors (Basel). 2023 Nov 29;23(23):9498. doi: 10.3390/s23239498. PMID: 38067871; PMCID: PMC10708748.
Swanepoel W. eHealth Technologies Enable more Accessible Hearing Care. Semin Hear. 2020 May;41(2):133-140. doi: 10.1055/s-0040-1708510. Epub 2020 Apr 7. PMID: 32269417; PMCID: PMC7138638.
Olawade DB, Wada OZ, Odetayo A, David-Olawade AC, Asaolu F, Eberhardt J. Enhancing mental health with artificial intelligence: Current trends and future prospects. J Med Surg Public Health [Internet]. 2024 Aug [cited 2024 Aug 10];3:100099. Available from: https://linkinghub.elsevier.com/retrieve/pii/S2949916X24000525
Zhao Y, Li J, Zhang M, Lu Y, Xie H, Tian Y, Qiu W. Machine Learning Models for the Hearing Impairment Prediction in Workers Exposed to Complex Industrial Noise: A Pilot Study. Ear Hear. 2019 May/Jun;40(3):690-699. doi: 10.1097/AUD.0000000000000649. PMID: 30142102; PMCID: PMC6493679.
Wang Y, Yao X, Wang D, Ye C, Xu L. A machine learning screening model for identifying the risk of high-frequency hearing impairment in a general population. BMC Public Health. 2024 Apr 25;24(1):1160. doi: 10.1186/s12889-024-18636-1. PMID: 38664666; PMCID: PMC11044481.
Jafleh EA, Alnaqbi FA, Almaeeni HA, Faqeeh S, Alzaabi MA, Al Zaman K. The Role of Wearable Devices in Chronic Disease Monitoring and Patient Care: A Comprehensive Review. Cureus. 2024 Sep 8;16(9):e68921. doi: 10.7759/cureus.68921. PMID: 39381470; PMCID: PMC11461032.
Pigini L, Bovi G, Panzarino C, Gower V, Ferratini M, Andreoni G, Sassi R, Rivolta MW, Ferrarin M. Pilot Test of a New Personal Health System Integrating Environmental and Wearable Sensors for Telemonitoring and Care of Elderly People at Home (SMARTA Project). Gerontology. 2017;63(3):281-286. doi: 10.1159/000455168. Epub 2017 Jan 19. PMID: 28099965.
Baig MM, Afifi S, GholamHosseini H, Mirza F. A Systematic Review of Wearable Sensors and IoT-Based Monitoring Applications for Older Adults - a Focus on Ageing Population and Independent Living. J Med Syst. 2019 Jun 15;43(8):233. doi: 10.1007/s10916-019-1365-7. PMID: 31203472.
Pan H, Lin Z, Fu P, Qi Y, Wang W. Modeling intra and inter-modality incongruity for multi-modal sarcasm detection. In: Findings of the Association for Computational Linguistics: EMNLP 2020 [Internet]. Online: Association for Computational Linguistics; 2020 [cited 2024 Dec 4]. p. 1383-92. Available from: https://www.aclweb.org/anthology/2020.findings-emnlp.124
Salamone F, Masullo M, Sibilio S. Wearable Devices for Environmental Monitoring in the Built Environment: A Systematic Review. Sensors (Basel). 2021 Jul 10;21(14):4727. doi: 10.3390/s21144727. PMID: 34300467; PMCID: PMC8309593.
Hearing Aid UK [Internet]. [cited 2024 Nov 26]. Can AI help your hearing? Available from: https://www.hearingaid.org.uk/hearing-aid-technology/how-artificial-intelligence-can-help-your-hearing
Saldana-Barrios JJ, Aguilar E, Ng W, Orocu R. Designing an IoT-Based System for Monitoring Noise Levels in the Computer Science Faculty and Library of the Technological University of Panama. Sensors (Basel). 2023 Nov 10;23(22):9083. doi: 10.3390/s23229083. PMID: 38005471; PMCID: PMC10675386.
Casillo M, Cecere L, Colace F, Lorusso A, Santaniello D. Integrating the Internet of Things (IoT) in SPA medicine: Innovations and challenges in digital wellness. Computers [Internet]. 2024 Mar 6 [cited 2024 Nov 26];13(3):67. Available from: https://www.mdpi.com/2073-431X/13/3/67
Ramadan MNA, Ali MAH, Khoo SY, Alkhedher M, Alherbawi M. Real-time IoT-powered AI system for monitoring and forecasting of air pollution in industrial environment. Ecotoxicol Environ Saf. 2024 Sep 15;283:116856. doi: 10.1016/j.ecoenv.2024.116856. Epub 2024 Aug 15. PMID: 39151373.
Wasmann JA, Lanting CP, Huinck WJ, Mylanus EAM, van der Laak JWM, Govaerts PJ, Swanepoel W, Moore DR, Barbour DL. Computational Audiology: New Approaches to Advance Hearing Health Care in the Digital Age. Ear Hear. 2021 Nov-Dec 01;42(6):1499-1507. doi: 10.1097/AUD.0000000000001041. PMID: 33675587; PMCID: PMC8417156.
Ganek HV, Madubueze A, Merritt CE, Bhutta ZA. Prevalence of hearing loss in children living in low- and middle-income countries over the last 10 years: A systematic review. Dev Med Child Neurol. 2023 May;65(5):600-610. doi: 10.1111/dmcn.15460. Epub 2022 Nov 17. PMID: 36397253.
Moroe NF. Early detection and management of occupational and environmental noise. In: Khoza-Shangase K, editor. Preventive audiology: An African perspective [Internet]. Cape Town: AOSIS; 2022 [cited 2024 Nov 26]. Available from: http://www.ncbi.nlm.nih.gov/books/NBK601304/
Patel H, Davy P, Tollemache C, Talbot N, Salmond J, Williams DE. Evaluating the efficacy of targeted traffic management interventions: A novel methodology for determining the composition of particulate matter in urban air pollution hotspots. Sci Total Environ. 2024 Nov 15;951:175414. doi: 10.1016/j.scitotenv.2024.175414. Epub 2024 Aug 8. PMID: 39127221.
Fabry DA, Bhowmik AK. Improving Speech Understanding and Monitoring Health with Hearing Aids Using Artificial Intelligence and Embedded Sensors. Semin Hear. 2021 Aug;42(3):295-308. doi: 10.1055/s-0041-1735136. Epub 2021 Sep 24. PMID: 34594091; PMCID: PMC8463124.
Lie A, Skogstad M, Johannessen HA, Tynes T, Mehlum IS, Nordby KC, Engdahl B, Tambs K. Occupational noise exposure and hearing: a systematic review. Int Arch Occup Environ Health. 2016 Apr;89(3):351-72. doi: 10.1007/s00420-015-1083-5. Epub 2015 Aug 7. PMID: 26249711; PMCID: PMC4786595.
Chen KH, Su SB, Chen KT. An overview of occupational noise-induced hearing loss among workers: epidemiology, pathogenesis, and preventive measures. Environ Health Prev Med. 2020 Oct 31;25(1):65. doi: 10.1186/s12199-020-00906-0. PMID: 33129267; PMCID: PMC7603754.
Hussein R, Griffin AC, Pichon A, Oldenburg J. A guiding framework for creating a comprehensive strategy for mHealth data sharing, privacy, and governance in low- and middle-income countries (LMICs). J Am Med Inform Assoc. 2023 Mar 16;30(4):787-794. doi: 10.1093/jamia/ocac198. PMID: 36259962; PMCID: PMC10018261.
Saeed SA, Masters RM. Disparities in Health Care and the Digital Divide. Curr Psychiatry Rep. 2021 Jul 23;23(9):61. doi: 10.1007/s11920-021-01274-4. PMID: 34297202; PMCID: PMC8300069.
Rejeb A, Rejeb K, Treiblmaier H, Appolloni A, Alghamdi S, Alhasawi Y, et al. The Internet of Things (IoT) in healthcare: Taking stock and moving forward. Internet of Things [Internet]. 2023 Jul 1;22:100721. Available from: https://www.sciencedirect.com/science/article/pii/S2542660523000446
Alahi MEE, Sukkuea A, Tina FW, Nag A, Kurdthongmee W, Suwannarat K, Mukhopadhyay SC. Integration of IoT-Enabled Technologies and Artificial Intelligence (AI) for Smart City Scenario: Recent Advancements and Future Trends. Sensors (Basel). 2023 May 30;23(11):5206. doi: 10.3390/s23115206. PMID: 37299934; PMCID: PMC10256108.
Valentin G, Nielsen CV, Nielsen AM, Tonnesen M, Bliksted KL, Jensen KT, Ingerslev K, Maribo T, Oestergaard LG. Bridging Inequity Gaps in Healthcare Systems While Educating Future Healthcare Professionals-The Social Health Bridge-Building Programme. Int J Environ Res Public Health. 2023 Sep 27;20(19):6837. doi: 10.3390/ijerph20196837. PMID: 37835107; PMCID: PMC10572531.
Lu L, Zhang J, Xie Y, Gao F, Xu S, Wu X, Ye Z. Wearable Health Devices in Health Care: Narrative Systematic Review. JMIR Mhealth Uhealth. 2020 Nov 9;8(11):e18907. doi: 10.2196/18907. PMID: 33164904; PMCID: PMC7683248.
FasterCapital [Internet]. [cited 2024 Dec 4]. The role of artificial intelligence in hearing solutions. Available from: https://fastercapital.com/keyword/the-role-of-artificial-intelligence-in-hearing-solutions.html
Clark H, Coll-Seck AM, Banerjee A, Peterson S, Dalglish SL, Ameratunga S, et al. A future for the world’s children? A WHO–UNICEF–Lancet Commission. Lancet [Internet]. 2020 Feb 22 [cited 2024 Nov 25];395(10224):605-58. Available from: https://doi.org/10.1016/S0140-6736(19)32540-1
Aslam B, Asghar R, Muzammil S, Shafique M, Siddique AB, Khurshid M, Ijaz M, Rasool MH, Chaudhry TH, Aamir A, Baloch Z. AMR and Sustainable Development Goals: at a crossroads. Global Health. 2024 Oct 17;20(1):73. doi: 10.1186/s12992-024-01046-8. PMID: 39415207; PMCID: PMC11484313
Dillard LK, Der CM, Laplante-Lévesque A, Swanepoel W, Thorne PR, McPherson B, de Andrade V, Newall J, Ramos HD, Kaspar A, Nieman CL, Clark JL, Chadha S. Service delivery approaches related to hearing aids in low- and middle-income countries or resource-limited settings: A systematic scoping review. PLOS Glob Public Health. 2024 Jan 24;4(1):e0002823. doi: 10.1371/journal.pgph.0002823. PMID: 38266001; PMCID: PMC10807760.