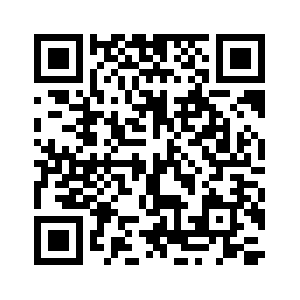
Knowledge Discovery on Artificial Intelligence and Physical Therapy: Document Mining Analysis
Physical Therapy受け取った 11 Nov 2024 受け入れられた 20 Nov 2024 オンラインで公開された 21 Nov 2024
Focusing on Biology, Medicine and Engineering ISSN: 2995-8067 | Quick Google Scholar
Next Full Text
Nanorobots in Medicine: Advancing Healthcare through Molecular Engineering: A Comprehensive Review
Previous Full Text
About the Problem of Climate Warming
受け取った 11 Nov 2024 受け入れられた 20 Nov 2024 オンラインで公開された 21 Nov 2024
Artificial intelligence (AI) is the simulation of human intelligence and benchmarks in Physical Therapy (PT). Therefore, the updated knowledge derived from large databases is highly engaging. Data Mining (DM) analysis from a big database related to “AI” and “PT” was the aim for the co-occurrence of words, network clusters, and trends under the Knowledge Discovery in Databases (KDD). The terms “AI” and “PT” were cited from a big database in the SCOPUS. The co-occurrence, network clustering, and trend were computer-analyzed with a Bibliometric tool. Between 1993 and 2024, 174 documents were published, revealing the most frequently used terms related to AI, human, PT, physical modalities, machine learning, physical treatment, deep learning, patient rehabilitation, robotics, virtual reality, algorithms, telerehabilitation, ergonomics, exercise, quality of life, and other related topics. Five network clusters were discovered as; (1) AI, decision support systems, health care, human-computer interaction, intelligent robots, learning algorithms, neuromuscular, stroke, and patient rehabilitation, PT, robotics, etc., respectively, (2) aged, algorithms, biomechanics, exercise, exercise therapy, female, humans, machine learning, middle-aged, PT modalities, rehabilitation, treatment outcome, deep learning, etc., (3) deep learning, diagnosis, and quality of life, (4) review and systematic review, and (5) clinical practice. From 2008 to 2024, a trend emerged in the fields of algorithms, computer-assisted diagnosis, treatment planning, classification, equipment design, signal processing, AI, exercise, physical and patient rehabilitation, robotics, virtual reality, machine learning, deep learning, clinical practice, etc. Discovered knowledge of AI with PT related to different machine learning for use in clinical practice.
Digital technology has been widely applied in healthcare, rehabilitation, and Physical Therapy (PT), with data science and analytics under statistics, operations research, data mining, computer science, databases, machine learning, mathematics, and distributed systems [
]. Presently, digital technology and Artificial Intelligence (AI) are currently encouraged by the American Physical Therapy Association (APTA) (2022) [ ], which enhances various advanced benefits in education, promotion, treatment, and rehabilitation [ ]. AI means the ability of computers to simulate human intellectual functions with decision support systems, machine learning, speech recognition, natural language processing, etc., [ ]. AI is often associated with cutting-edge technology, which has been advancements in a particular branch of the subject called "Machine Learning (ML)," where creativity has given rise to what is referred to as "Deep Learning" (DL), as well as the application of AL, ML, and DL in innovative robotics on the different algorithms such as ensemble algorithms, decision trees, instance-based algorithms, and artificial neural networks [ ]. Previous evidence supports that AI-based technologies have become more important in supplementing cognitive skills in humans and clinical decision-making [ ]. However, a previous report found that PT’s knowledge of AI applications in rehabilitation was lower than general AI technology [ ]. Therefore, innovative knowledge or information on AI for PT should be evaluated under scientific methods and specific algorithms [ ]. Healthcare settings can use AI systems' sophisticated algorithms and self-learning capabilities to improve workflow efficiency, risk assessment models, and diagnosis accuracy [ ]. Especially, AI-based technology has been challenged in various healthcare as well as physical rehabilitation relating to physical function, activity, pain, and health-related quality [ ] either clinical practice or education [ ]. Regrettably, we cannot confirm the scope and trend of AI on PT across different technologies, procedures, or target populations, but it warrants further study. Therefore, it is necessary to perform information extraction from documents in databases. Data mining analysis is one of many scientific methods that can be used to discover knowledge or information, which is called “Knowledge Discovery in Databases” (KDD) [ ]. KDD has been studied by a large number of academics in the fields of business, industry, social media, and medicine [ ] with advanced statistical techniques [ ] and tools, especially a Bibliometric tool [ ]. The benefits of this tool include the ability to identify and present data sources and relative word networks, cluster many keywords, and track keyword trends in big text data using specific algorithms, as demonstrated in a previous study [ ]. Therefore, an advantage of the results from text data mining analysis is the widespread understanding of the informative changes related to AI and PT, which was the aim of this study.This study involved conducting a data mining analysis to uncover knowledge within a database, a process known as Knowledge Discovery in Databases (KDD). Many academics in the fields of business, industry, social media, and medicine have applied this rapidly emerging and transdisciplinary discipline [
]. DM techniques can uncover information and important features in a dataset [ ].The manual examination and interpretation of data is the foundation of the conventional approach to turning data into knowledge. Prediction through (1) classification, regression identification, and grouping, as well as (2) description, are typically the two high-level principal goals of data mining in practice. Model representation, model assessment, and search are used, correspondingly, to develop the various parts of data mining algorithms. The basic theory of the KDD process includes selecting data from databases or data warehouses, preprocessing and cleaning the data, and then transforming it into the data mining process [
]. Following the completion of DM, data will be interpreted and evaluated to ensure optimal performance for getting a new idea or concept. Numerous research domains employ bibliometric analysis as one of several processes, utilizing both data and text mining. From 2009 to 2018, researchers studied DM and ML techniques in public health using a variety of tools [ ], mirroring the findings of an updated study on "virtual reality" and "pulmonary rehabilitation" in 2024 [ ]. In DM, there are four stages to the bibliometric analysis: 1) organizing the review procedure, 2) gathering data, 3) performing the bibliometric analysis, and 4) summarizing the report. This study employs a text data mining analysis with the Bibliometric analysis under RStudio Software (version 4.3.2) from the SCOPUS database in steps 1 to 5 as Figure 1, followed by a previous study [ ].The selection criteria were concluded with (1) specific keywords of “Artificial Intelligence (AI)” and “Physical Therapy (PT)”, (2) the published documents were cited on the SCOPUS database conducted between 1993 and 2024, and (3) with literature type either final or article in press, conference papers, articles, reviews, editorials, book chapters, books, errata, letters, and notes. Data filtering was defined in the English language. Data was downloaded and saved as .CVS file. The retrieved data were then exported to an Excel file in the Bibliometric tool can be used to analyze scientific domains [
] and the data was formatted before integrating from other databases with Biblioshiny. In addition, the filtering process was performed after integrating completely in Biblioshiny by removing irrelevant and duplicate documents.After data collection and export from the SCOPUS database in steps I and II, data processing in the Bibliometric method within RStudio Software in step III. The data was then normalized using Salton's Cosine algorithm (step IV) [
].The primary most relevant sources or journals, number of documents, keyword occurrences or most frequency words, network clustering, and trend were analyzed using the Walktrap distance algorithm [
]. The Kamada-Kawai layout algorithm was used to represent the data as a mapping visualization based on each weight and distance between all words [ ].From 1993 to 2024, we cited 174 documents related to AI and PT from the SCOPUS database. All documents were published in English under 168 final and 6 articles in press status. All documents consisted of 75 conference papers, 73 articles, 14 reviews, 4 editorials, 2 book chapters, 2 books, 2 errata, 1 letter, and 1 note. In all documents, there were different affinities of authors published by the Department of Engineering, Computer Sciences, Computing Engineering, Sciences, Electronics, Health Informatics, etc. There were a few documents published by the Department of Physical Therapy (12 documents), Rehabilitation Medicine (10 documents), and other Departments of Medicine.
A total of 174 documents with “Artificial Intelligence” and “Physical Therapy” were found between 1993 and 2024 from 137 sources. From 1993, 2003, and 2005, the first document appeared, followed by two yearly publications from 2006 to 2011. After 2012, more published documents were presented until 2019. Rapidly published documents were found in 2020 (n = 17), 2021 (n = 11), 2023 (n = 29), and 2024 (n = 38), respectively. The relevant sources or journals were published those documents; Lecture Notes in Computer Science (n = 11), Sensors (n = 5), Studies in Health Technology and Informatics (n = 4), IEEE Transactions on Neural Systems and Rehabilitation Engineering (n = 3), Musculoskeletal Science and Practice (n = 3), ACM International Conference Proceeding Series (n = 2), Advances in Intelligent systems and Computing (n = 2), Artificial Intelligent in Medicine (n = 2), Frontiers in Public Health (n = 2), IEEE Access (n = 2), IEEE Sensors Journal (n = 2), International Journal of Environmental Research and Public Health (n = 2), Journal of Medical Internet Research (n = 2), Journal of Neuroengineering and Rehabilitation (n = 2), Journal of Physics: Conference Series (n = 2), Lecture Notes in Electrical Engineering (n = 2), Lecture Notes in Networks and Systems (n = 2), Physical Therapy (n = 2), Proceedings of the Human Factors and Ergonomics Society (n = 2), Progress in Biomedical Optics and Imaging-Proceedings of Spie (n = 2), and Sensors (Switzerland) (n = 2), etc.
The result of the bibliometric analysis of 174 documents, the most frequent words in those documents showed in Figure 2; artificial intelligence (n = 163, 16%), human (n = 68, 6%), physical therapy (n = 64, 6%), physiotherapy (n = 51, 5% ), humans (n = 50, 5%), article (n = 41, 4%), female (n = 38, 4%), male (n = 38, 4%), physical therapy modalities (n = 33, 3%), machine learning (n = 32, 3%), adult (n = 30, 3% ), patient treatment (n = 27, 3% ), deep learning (n = 22, 2%), patient rehabilitation (n = 22, 2%), robotics (n = 19, 2%), rehabilitation (n = 18, 2%), virtual reality (n = 18, 2%), algorithm (n = 17, 2%), algorithms (n = 17, 2%), controlled study (n = 15,1%), learning systems (n = 15, 1%), procedures (n = 13, 1%), aged (n = 12, 1%), middle aged (n = 12, 1%), treatment outcome (n = 12, 1%), physiotherapist (n = 10, 1%), stroke rehabilitation (n = 10, 1%), health care (n = 9, 1%), ergonomics (n = 8, 1%), exercise (n = 8, 1%), intelligent robots (n = 8, 1%), quality of life (n = 8, 1%), systematic review (n = 8, 1%), telerehabilitation (n = 8, 1%), biomechanics (n = 7, 1%), diagnosis (n = 7, 1%), exercise therapy (n = 7, 1%), gait (n = 7, 1%), natural language processing (n = 7, 1%), etc., respectively.
The co-occurrence networks of various sets were displayed in Figure 3 as a result of the network approach analysis. The first network included artificial intelligence, deep learning, algorithms, procedures, methodology, computer vision, sensitivity and specificity, quality of life, systematic review, and review. The second network encompassed physical therapy, learning systems, virtual reality, learning algorithms, patient treatment, robotics, health care, ergonomics, decision support systems, ergonomics, diagnosis, and human-computer interactions. The last network included physiotherapy, human, human, physiotherapist, exercise, biomechanics, clinical practice, gait, treatment outcome, telerehabilitation, natural language processing, aged, middle-aged, controlled study, female, male, adult, and major clinical study.
The results of cluster analysis showed five clusters in Table 1 and Figure 4. The first cluster was composed of fifteen words of artificial intelligence, decision support systems, health care, human-computer interaction, intelligent robots, learning algorithms, learning systems, muscle, neuromuscular rehabilitation, patient rehabilitation, patient treatment, physical therapy, robotics, stroke rehabilitation, and virtual reality. The second cluster consisted of adult, aged, algorithm, algorithms, article, biomechanics, controlled study, exercise, exercise therapy, female, human, humans, machine learning, major clinical study, male, methodology, middle-aged, physical therapy modalities, physiotherapist, priority journal, rehabilitation, rehabilitation, and treatment outcome. The third cluster consisted of deep learning, diagnosis, and quality of life. The last cluster was clinical practice.
The words’ frequency analysis showed an progressive trend from 2008 to 2024 as shown in Figure 5; pattern recognition (n = 5) in 2008 to 2013, automated pattern recognition (n = 6) in 2008 to 2013, and methodology (n = 8) in 2008 to 2013, treatment planning (n = 5) in 2010 to 2024, algorithms (n = 17) in 2008 to 2018, classification (n = 5) in 2013 to 2024, computer assisted diagnosis (n = 5) in 2008 to 2013, sensitivity and specificity (n = 10) in 2008 to 2013, physiology (n = 5) in 2014 to 2015, diseases (n = 5) and equipment design (n = 5) in 2014 to 2015, shoulder (n = 5) in 2015 to 2022, physical rehabilitation (n = 5) in 2015 to 2017, signal processing (n = 5) in 2014 to 2022, patient rehabilitation (n = 22) in 2015 to 2021, rehabilitation exercise (n = 5) in 2015 to 2022, decision support system (n = 9) in 2014 to 2019, learning systems (n = 15) in 2014 to 2023, human computer interaction (n = 7) in 2016 to 2022, exercise (n = 8) in 2014 to 2024, priority journal (n = 11) in 2015 to 2019, algorithm (n = 17) in 2011 to 2021, virtual reality (n = 18) in 2016 to 2023, physical therapy (n = 64) in 2015 to 2023, procedures (n = 13) and robotics (n = 19) in 2015 to 2022, artificial intelligence (n = 163) in 2014 to 2023, female (n = 38) in 2016 to 2024, male (n = 38) in 2015 to 2023, article (n = 41) in 2013 to 2023, humans (n = 50) in 2018 to 2023, physiotherapy (n = 51) in 2020 to 2024, human (n = 68) in 2019 to 2024, aged (n = 12) in 2022 to 2024, deep learning (n = 22) in 2022 to 2024, machine learning (n = 32) in 2020 to 2024, forecasting (n = 5) in 2023 to 2024, clinical practice (n=8) in 2020 to 2024, and review (n = 8) in 2022 to 2024, respectively.
This study was run on the text data mining analysis related to two keywords of AI and PT with the Bibliometric tool in the RStudio program. This tool is popularly used because of standardized software product lines in engineering with low cost, short time, and high quality [
]. This tool also has been applied in other fields such as business, medicine, and education from scientific databases such as SCOPUS [ ], to discover novel knowledge in various fields [ ]. Moreover, many advantages of data mining by Bibliometric tools show repeatability in a broad scope from either small or big datasets from the globally published articles in SCOPUS or PubMed both quantitative and qualitative results such as co-occurrence, network clustering, and trend compared to other qualitative analysis in meta-analysis, and systematic literature review.Data mining and knowledge discovery in databases (KDD), a rapidly developing and transdisciplinary discipline [
]), can apply this in diverse fields such as business, industry, social media, and medicine [ ]. Thus, data mining, also known as KDD, is an advanced statistical technique that identifies previously unknown patterns [ ]. The manual examination and interpretation of data is the foundation of the conventional approach to turning data into knowledge. Prediction through (1) classification, regression identification, and grouping, as well as (2) description, are typically the two high-level principal goals of data mining in practice. Accordingly, we use model representation, model assessment, and search to develop the various parts of data-mining algorithms [ ].According to Brachman and Anand (1996) [
], the KDD process consists of the following fundamental steps: (1) establishing a goal and gaining an understanding of the application domain; (2) cleaning and preprocessing data; (3) data reduction; (5) matching the goals to a specific DM method; (6) exploratory analysis and model and hypothesis selection; (7) DM by looking for patterns of interest in a specific representation form or a set of such representations, including classification rules or trees, regression, and clustering; (8) interpreting mined patterns and acting on the knowledge discovered in the final step. Therefore, the Bibliometric tool is a computer analysis for identifying new knowledge that is reflected as a great tool with reliable, clear, and unbiased quantitative as the previous suggestion [ ].However, as a property of documents from Scopus in this study, it is relatively more comprehensive than PubMed or Web of Science (WoS) because data in Scopus covered 84% of the WoS titles [
]. Furthermore, Scopus surpasses Google Scholar in precision due to its superior control over referred publications, controlled indexing [ ], accessibility, and extensive coverage of peer-reviewed academic literature [ ]. Therefore, the SCOPUS database provided interesting big data related to the keywords "AI" and "PT", which were cited to identify co-occurrence, network clusters, and future trends.Before co-occurrence and network clustering evaluation, the validity of the data was pre-screened with the Cosine normalization algorithm as previous evidence [
]. The cosine normalization algorithm is a statistical data science tool that has been used instead of the Dot Product in Neural Networks analysis by the Euclidean distance method [ ]. This algorithm can achieve lower test error and is more stable than batch, weight, and layer normalization techniques. When the data was optimized for normalization, the co-occurrence was identified under the Kamada & Kawai algorithm. This algorithm was standardized and applied to identify the co-occurrence words from other databases such as PubMed [ ] that referred to the probabilistic model for detecting statistically significant pairwise co-occurred terms.The network approach analysis revealed three dominant co-occurrence networks of different sets with co-occurrence matrixes [
]. The first network was artificial intelligence, deep learning, algorithms, procedures, methodology, computer vision, sensitivity and specificity, quality of life, systematic review, and review. The second network was made up of physical therapy, learning systems, virtual reality, learning algorithms, patient treatment, robotics, health care, ergonomics, decision support systems, ergonomics, diagnosis, intelligence robots, muscle, and human-computer interactions. The last network included physiotherapy, human, human, physiotherapist, exercise, biomechanics, clinical practice, gait, treatment outcome, telerehabilitation, natural language processing, aged, middle-aged, controlled study, female, male, adult, and major clinical study. This data was visualized using the Kamada & Kawai algorithm [ ].The Walktrap algorithm identified the final method for network clustering in this study, discovering five clusters. The first cluster was composed of fifteen words of artificial intelligence, decision support systems, health care, human-computer interaction, intelligent robots, learning algorithms, learning systems, muscle, neuromuscular rehabilitation, patient rehabilitation, patient treatment, physical therapy, robotics, stroke rehabilitation, and virtual reality. The second cluster consisted of adult, aged, algorithm, algorithms, article, biomechanics, controlled study, exercise, exercise therapy, female, human, humans, machine learning, major clinical study, male, methodology, middle-aged, physical therapy modalities, physiotherapist, priority journal, rehabilitation, rehabilitation, and treatment outcome. The third cluster consisted of deep learning, diagnosis, and quality of life. The fourth cluster encompassed reviews and systematic reviews, while the final cluster was focused on clinical practice. A previous study used the basic theory of cluster analysis to determine the dimension or centrality scale between words situated in four quadrants [
].Furthermore, the Walktrap algorithm in this study [
] relies on a hierarchical agglomerative clustering process that generates the concept of short-distance random walks on the network over an extended period [ ], which corresponds to the period from 1993 to 2024 in this study. Finally, this study's network clustering visualization aligns with various network algorithms like Edge, Betweenness Fast Greedy, Leading Eigenvector, Infomap, Label Propagation, and Louvain [ ]. Finally, the co-occurrence and network clusters of all text data revealed intriguing results pertaining to the challenging research platform related to AI and PT from 2008 to 2024.The previous study, which reviewed the document database in Web of Science (WoS) from 2014 to 2023, revealed that rehabilitation, recovery, and stroke were the most frequently used keywords across 1,216 research documents [
]. However, the result didn't identify the trend and clustering words that presented the future research plans and trends. This study was identified in a wider published period from 2008 to 2024 that showed interesting keywords from data mining analysis; for example, pattern recognition, automated pattern recognition, treatment planning, algorithms, classification, computer-assisted diagnosis, physiology, diseases, equipment design, shoulder, physical rehabilitation, signal processing, patient rehabilitation, rehabilitation exercise, decision support system, learning systems, human-computer interaction, exercise, virtual reality, physical therapy, robotics, artificial intelligence, female, male, physiotherapy, human, deep learning, machine learning, forecasting, clinical practice, and review. Furthermore, the trend results from this study are consistent with a previous review report that proposed deep learning models in machine learning involving various PT roles such as medical tests and functional movement screening [ ], post-stroke exercise [ ], as well as human gait teaching [ ]. The next generation of PT has rapidly applied AI-based technology and ML; for example, the ViFive mobile application used AI to measure the accuracy of vision on a range of motion (ROM) [ ]. Data on the accuracy of AI-based technology for healthcare educational tools shows that ChatGPT-4 and Microsoft Copilot outperformed Google Gemini [ ]. AI and ML were applied to classify fall risks from time-up-and-go (TUG) scores in community-dwelling older adults with the aid of an explainable AI [ ]. In addition, the updated review showed the ML efficiently processes large datasets enhanced in clinical practice for PT with 14 publications in musculoskeletal PT, 9 publications in neurological PT, and 8 documents in sports PT that related to diagnosis, prognosis, treatment outcome prediction, clinical decision support system, movement analysis, patient monitoring, and personalized care plan [ ]. This result supports the updated report that documented the transformative potential of AI in healthcare [ ] and patient care [ ]. Thus, it can be suggested that AI will play various roles in supporting clinical practice in different PT fields such as musculoskeletal, cardiopulmonary, neurological, gerontology, and sports in the future.Therefore, this discovered knowledge on databases on AI and PT from data mining analysis with bibliometric tools can be encouraged in exercise and rehabilitation under machine learning with classification, deep learning, forecasting, decision support systems, signal processing, or computer-assisted systems in humans, especially for forecasting, testing, and medical screening that can be the guideline in clinical practice guideline in the future. For instance, the ViFive AI mobile application was used to assess the range of motion of the shoulder, hip, knee, and ankle in patients and healthy adults as part of the use of AI in clinical practice. This then revealed a substantial correlation with the accuracy of the therapist's measurement [
]. This suggests that in the future, clinical practice may make use of AI technology. Although AI is incredibly challenging to revolutionize physical therapy practices, ethical considerations should ensure the responsiveness of the legal and equitable application of AI tools.This data was cited from the SCOPUS database, English literature, and all types of documents on the keywords “Artificial Intelligence” and “Physical therapy”, which were both final and articles in press. There are other published databases, such as Web of Science (WoS), and other platforms that recruit other words. These possibly show more words, influence on co-occurrence, network, and trend topic in this result. Therefore, future research should focus more on robust evidence of its usefulness.
van der Aalst W. Process mining-Data science in action. 2nd ed. Springer; 2016.
American Physical Therapy Association (APTA). The digital enabled physical therapist: an APTA foundational paper. November 2022. https://www.apta.org/contentassets/e37aa1765cab4b1791d22717d3ac20af/apta-digital-health-foundational-paper-2022.pdf
Kahie M, Deshmukh N, Makhija LH, Chaudhary S, Ambad R, Bankar N. Artificial intelligence (AI) and machine learning (ML) in clinical practice and physiotherapy. Ann Med Health Sci Res. 2021;11(S3):158-159.
Sheikh H, Prins C, Schrijvers E. Chapter 2 - Artificial Intelligence: definition and background. In: Mission AI. 2023. p. 15-41. doi: 10.1007/978-3-031-21448-6_2.
Soori M, Arezoo B, Dastres R. Artificial intelligence, machine learning and deep learning in advanced robotics: a review. Cogn Robot. 2023;3:54-70.
Harwich E, Laycock K. Thinking on its own: AI in the NHS. London Reform; 2018. http://www.reform.uk/wp-content/uploads/2018/01/AI-in-Healthcare-report.pdf
Alsobhi M, Khan F, Chevidikunnan MF, Basuodan R, Shawli L, Neamatallah Z. Physical Therapists' Knowledge and Attitudes Regarding Artificial Intelligence Applications in Health Care and Rehabilitation: Cross-sectional Study. J Med Internet Res. 2022 Oct 20;24(10):e39565. doi: 10.2196/39565. PMID: 36264614; PMCID: PMC9634519.
Kudande D, Shah BN. A review paper on formation of data science and fundamentals. Inter J Engin App Sci Technol 2020:4(2):150-152.
Kaul V, Enslin S, Gross SA. History of artificial intelligence in medicine. Gastrointest Endosc. 2020 Oct;92(4):807-812. doi: 10.1016/j.gie.2020.06.040. Epub 2020 Jun 18. PMID: 32565184.
Sumner J, Lim HW, Chong LS, Bundele A, Mukhopadhyay A, Kayambu G. Artificial intelligence in physical rehabilitation: A systematic review. Artif Intell Med. 2023 Dec;146:102693. doi: 10.1016/j.artmed.2023.102693. Epub 2023 Nov 2. PMID: 38042593.
Mahto RK, Kumari A, Humari S. The use of AI in physical therapy. Educ Adm Theory Pract. 2024;30(4):5694-5696.
Upadhyay A. A Review on Bibliometric Analysis of Data Mining. Int J Eng Res Comp Sci Eng. 2018;5(4):621-615.
Fayyad U, Piatetsky-Shapiro G, Smyth P. From Data Minning to Knowledge Discovery in Databases. Am Assoc Artif Intell. 1996;17(3): 37-53.
Choudhri AF, Siddiqui A, Khan NR, Cohen HL. Understanding bibliometric parameters and analysis. Radiographics. 2015 May-Jun;35(3):736-46. doi: 10.1148/rg.2015140036. PMID: 25969932.
Dissanayake K, Johar MGM, Ubeysekara NH. Data mining techniques in disease classification: descriptive bibliometric analysis and visualization of global publications. Int J Comp Digital Sys. 2023;13(1):289-301.
Leelarungrayub J, Chantaraj P, Thipcharoen S, Jintana J. Updated research trend and clustering algorithm on virtual reality and pulmonary rehabilitation: Scopus-based bibliometric and visual analysis. J Assoc Med Sci. 2024;57(2):173-182.
Cobo MJ, Lopez-Herrera AG, Herrera-Viedma E, Herrera F. Science mapping software tools: review, analysis, and cooperative study among tools. J Am Soc Inform Sci Technol. 2021;62(7):1382-1402.
Leydesdorff L. On the normalization and visualization of author co-citation data: Salton’s Cosine versus the Jaccard Index. J Am Soc Inform Sci Technol. 2008;59(1):77-85.
Pons P, Latapy M. Computing communities in large networks using random walks. In: Yolum P, Güngör T, Gürgen F, Özturan C, editors. Computer and Information Sciences - ISCIS 2005. Berlin, Heidelberg: Springer; 2005. p. 284-293.
Kamada T, Kawai S. An algorithm for drawing general undirected graphs. Inf Proc Lett. 1989;49(11-12):7-15.
Heradio R, Perez-Morago H, Fernandez-Amoros D, Cabrerizo FJ, Herrera-Viedma E. A bibliometric analysis of 20 years of research on software product lines. Inf Softw Technol. 2016;72:1-15.
Kang GJ, Ewing-Nelson SR, Mackey L, Schlitt JT, Marathe A, Abbas KM, Swarup S. Semantic network analysis of vaccine sentiment in online social media. Vaccine. 2017 Jun 22;35(29):3621-3638. doi: 10.1016/j.vaccine.2017.05.052. Epub 2017 May 27. PMID: 28554500; PMCID: PMC5548132.
Donthu N, Kumar S, Mukherjee D, Pandey N, Lim WM. How to conduct a bibliometric analysis: an overview and guidelines. J Bus Res. 2021;133:285-296.
Joshi S, Nair MK. Prediction of heart disease using classification-based data mining technique. In: Computational Intelligence in Data Mining-Volume 2. Springer; 2015;503-511.
Brachman RJ, Anand T. The process of knowledge discovery in databases: a human-centered approach. In: Advances in Knowledge Discovery and Data Mining. Menlo Park: AAAI Press/The MIT Press; 1996;37-58.
Kokol P, Blažun Vošner H, Završnik J. Application of bibliometrics in medicine: a historical bibliometrics analysis. Health Info Libr J. 2021 Jun;38(2):125-138. doi: 10.1111/hir.12295. Epub 2020 Jan 29. PMID: 31995273.
Garrido-Cardenas JA, de Lamo-Sevilla C, Cabezas-Fernández MT, Manzano-Agugliaro F, Martínez-Lirola M. Global tuberculosis research and its future prospects. Tuberculosis (Edinb). 2020 Mar;121:101917. doi: 10.1016/j.tube.2020.101917. Epub 2020 Feb 23. PMID: 32279873.
Dinic B, Jevremov T. Trends in research related to the dark triad: a bibliometric analysis. Curr Psychol. 2021;40(7):3206-3215.
Mongeon P, Paul-Hus A. The journal coverage of Web of Science and Scopus: a comparative analysis. Scientometrics. 2016;106(1):213-228.
Chunjie L, Jianfeng Z, Lei W, Qiang Y. Cosine normalization: using cosine similarity instead of dot product in neural networks. arXiv. 2017. https://doi.org/10.48550/arXiv.1702.05870.
Zhou X, Zhou M, Huang D, Cui L. A probabilistic model for co-occurrence analysis in bibliometrics. J Biomed Inform. 2022 Apr;128:104047. doi: 10.1016/j.jbi.2022.104047. Epub 2022 Mar 4. PMID: 35257868.
Luukkonen T, Tijssen R, Persson ORT, Sivertsen G. The measurement of international scientific collaboration. Scientometrics. 1993;28(1):15-36.
Kobourov SG. Force-directed drawing algorithms. In: Tamassia R, editor. Handbook of graph drawing and visualization. New York: CRC Press; 2013;383-408.
Yang K, Wang L, Yang G, Jiang X. Research hotspots and trends in nursing education from 2014 to 2020: A co-word analysis based on keywords. J Adv Nurs. 2022 Mar;78(3):787-798. doi: 10.1111/jan.15010. Epub 2021 Sep 12. PMID: 34514616.
de Rezende LB, Blackwell P, Goncalves M. Research focuses, trends, and major findings on project complexity: a bibliometric network analysis of 50 years of project complexity research. Proj Manage J. 2018;49(1):42-49.
Lee Y, Lee Y, Seong G, Stanescu A, Hwang CS. Analysis of GIS research trends in the US with AAG conference presentations from 2000-2019. Geogr J Korea. 2019;53(4):495-508.
Subbarayalu AV, Idhris M, Prabaharan S, Kamalasanan A, Samuel SA, Sakthivel M, Pattukuthu A, Pandiyarajan A, Ali MB, Purushothaman VK, Marimuthu PR. Research trends in the application of artificial intelligence in physical therapy rehabilitation: a bibliometric study. Acta Biomed. 2024;95(2):e2024087.
Nogales A, Rodríguez-Aragón M, García-Tejedor ÁJ. A systematic review of the application of deep learning techniques in the physiotherapeutic therapy of musculoskeletal pathologies. Comput Biol Med. 2024 Apr;172:108082. doi: 10.1016/j.compbiomed.2024.108082. Epub 2024 Jan 29. PMID: 38461697.
Murikipudi M, Azmee AA, Khan MAAH, Pei Y. CBSA: a deep transfer learning framework for assessing post-stroke exercises. In: Proceedings-2024 IEEE/ACM Connected Health: Applications, Systems and Engineering Technologies. Chase; 2024;85-96. doi: 10.1109/CHASE60773.2024.00018.
Lowe SW. The use of artificial intelligence in crafting a novel method for teaching normal human gait. Eur J Physiother. 2024;1-5.
Meng Y, Lee M, Clark HJY, Nguyen C, Kwon S, Schultz S, Pham NS, Schirmer D, Roh EY. Evaluation of range of motion using vision artificial intelligence (AI) in musculoskeletal medicine. J Med Artif Intell. 2024;7:22.
Rossettini G, Rodeghiero L, Corradi F, Cook C, Pillastrini P, Turolla A, Castellini G, Chiappinotto S, Gianola S, Palese A. Comparative accuracy of ChatGPT-4, Microsoft Copilot and Google Gemini in the Italian entrance test for healthcare sciences degrees: a cross-sectional study. BMC Med Educ. 2024 Jun 26;24(1):694. doi: 10.1186/s12909-024-05630-9. PMID: 38926809; PMCID: PMC11210096.
Liang HW, Ameri R, Band S, Chen HS, Ho SY, Zaidan B, Chang KC, Chang A. Fall risk classification with posturographic parameters in community-dwelling older adults: a machine learning and explainable artificial intelligence approach. J Neuroeng Rehabil. 2024 Jan 29;21(1):15. doi: 10.1186/s12984-024-01310-3. PMID: 38287415; PMCID: PMC10826018.
Reis FJJ, Carvalho MBL, Neves GA, Nogueira LC, Meziat-Filho N. Machine learning methods in physical therapy: A scoping review of applications in clinical context. Musculoskelet Sci Pract. 2024 Sep 13;74:103184. doi: 10.1016/j.msksp.2024.103184. Epub ahead of print. PMID: 39278141.
Bekbolatova M, Mayer J, Ong CW, Toma M. Transformative Potential of AI in Healthcare: Definitions, Applications, and Navigating the Ethical Landscape and Public Perspectives. Healthcare (Basel). 2024 Jan 5;12(2):125. doi: 10.3390/healthcare12020125. PMID: 38255014; PMCID: PMC10815906.
Shawli L, Alsobhi M, Faisal Chevidikunnan M, Rosewilliam S, Basuodan R, Khan F. Physical therapists' perceptions and attitudes towards artificial intelligence in healthcare and rehabilitation: A qualitative study. Musculoskelet Sci Pract. 2024 Oct;73:103152. doi: 10.1016/j.msksp.2024.103152. Epub 2024 Jul 24. PMID: 39067366.
Leelarungrayub J, Yankai A, Thipcharoen S. Knowledge Discovery on Artificial Intelligence and Physical Therapy: Document Mining Analysis. IgMin Res. November 21, 2024; 2(11): 929-937. IgMin ID: igmin270; DOI:10.61927/igmin270; Available at: igmin.link/p270
次のリンクを共有した人は、このコンテンツを読むことができます:
1Department of Physical Therapy, Faculty of Associated Medical Sciences, Chiang Mai University, Chiang Mai 50200, Thailand
2Department of Data Science and Digital Innovation, Faculty of Innovation Technology and Creativity, The Far Eastern University, Chiang Mai, 50100 Thailand
Address Correspondence:
Jirakrit Leelarungrayub, Department of Physical Therapy, Faculty of Associated Medical Sciences, Chiang Mai University, Chiang Mai 50200, Thailand, Email: [email protected], [email protected]
How to cite this article:
Leelarungrayub J, Yankai A, Thipcharoen S. Knowledge Discovery on Artificial Intelligence and Physical Therapy: Document Mining Analysis. IgMin Res. November 21, 2024; 2(11): 929-937. IgMin ID: igmin270; DOI:10.61927/igmin270; Available at: igmin.link/p270
Copyright: © 2024 Leelarungrayub L, et al. This is an open access article distributed under the Creative Commons Attribution License, which permits unrestricted use, distribution, and reproduction in any medium, provided the original work is properly cited.
van der Aalst W. Process mining-Data science in action. 2nd ed. Springer; 2016.
American Physical Therapy Association (APTA). The digital enabled physical therapist: an APTA foundational paper. November 2022. https://www.apta.org/contentassets/e37aa1765cab4b1791d22717d3ac20af/apta-digital-health-foundational-paper-2022.pdf
Kahie M, Deshmukh N, Makhija LH, Chaudhary S, Ambad R, Bankar N. Artificial intelligence (AI) and machine learning (ML) in clinical practice and physiotherapy. Ann Med Health Sci Res. 2021;11(S3):158-159.
Sheikh H, Prins C, Schrijvers E. Chapter 2 - Artificial Intelligence: definition and background. In: Mission AI. 2023. p. 15-41. doi: 10.1007/978-3-031-21448-6_2.
Soori M, Arezoo B, Dastres R. Artificial intelligence, machine learning and deep learning in advanced robotics: a review. Cogn Robot. 2023;3:54-70.
Harwich E, Laycock K. Thinking on its own: AI in the NHS. London Reform; 2018. http://www.reform.uk/wp-content/uploads/2018/01/AI-in-Healthcare-report.pdf
Alsobhi M, Khan F, Chevidikunnan MF, Basuodan R, Shawli L, Neamatallah Z. Physical Therapists' Knowledge and Attitudes Regarding Artificial Intelligence Applications in Health Care and Rehabilitation: Cross-sectional Study. J Med Internet Res. 2022 Oct 20;24(10):e39565. doi: 10.2196/39565. PMID: 36264614; PMCID: PMC9634519.
Kudande D, Shah BN. A review paper on formation of data science and fundamentals. Inter J Engin App Sci Technol 2020:4(2):150-152.
Kaul V, Enslin S, Gross SA. History of artificial intelligence in medicine. Gastrointest Endosc. 2020 Oct;92(4):807-812. doi: 10.1016/j.gie.2020.06.040. Epub 2020 Jun 18. PMID: 32565184.
Sumner J, Lim HW, Chong LS, Bundele A, Mukhopadhyay A, Kayambu G. Artificial intelligence in physical rehabilitation: A systematic review. Artif Intell Med. 2023 Dec;146:102693. doi: 10.1016/j.artmed.2023.102693. Epub 2023 Nov 2. PMID: 38042593.
Mahto RK, Kumari A, Humari S. The use of AI in physical therapy. Educ Adm Theory Pract. 2024;30(4):5694-5696.
Upadhyay A. A Review on Bibliometric Analysis of Data Mining. Int J Eng Res Comp Sci Eng. 2018;5(4):621-615.
Fayyad U, Piatetsky-Shapiro G, Smyth P. From Data Minning to Knowledge Discovery in Databases. Am Assoc Artif Intell. 1996;17(3): 37-53.
Choudhri AF, Siddiqui A, Khan NR, Cohen HL. Understanding bibliometric parameters and analysis. Radiographics. 2015 May-Jun;35(3):736-46. doi: 10.1148/rg.2015140036. PMID: 25969932.
Dissanayake K, Johar MGM, Ubeysekara NH. Data mining techniques in disease classification: descriptive bibliometric analysis and visualization of global publications. Int J Comp Digital Sys. 2023;13(1):289-301.
Leelarungrayub J, Chantaraj P, Thipcharoen S, Jintana J. Updated research trend and clustering algorithm on virtual reality and pulmonary rehabilitation: Scopus-based bibliometric and visual analysis. J Assoc Med Sci. 2024;57(2):173-182.
Cobo MJ, Lopez-Herrera AG, Herrera-Viedma E, Herrera F. Science mapping software tools: review, analysis, and cooperative study among tools. J Am Soc Inform Sci Technol. 2021;62(7):1382-1402.
Leydesdorff L. On the normalization and visualization of author co-citation data: Salton’s Cosine versus the Jaccard Index. J Am Soc Inform Sci Technol. 2008;59(1):77-85.
Pons P, Latapy M. Computing communities in large networks using random walks. In: Yolum P, Güngör T, Gürgen F, Özturan C, editors. Computer and Information Sciences - ISCIS 2005. Berlin, Heidelberg: Springer; 2005. p. 284-293.
Kamada T, Kawai S. An algorithm for drawing general undirected graphs. Inf Proc Lett. 1989;49(11-12):7-15.
Heradio R, Perez-Morago H, Fernandez-Amoros D, Cabrerizo FJ, Herrera-Viedma E. A bibliometric analysis of 20 years of research on software product lines. Inf Softw Technol. 2016;72:1-15.
Kang GJ, Ewing-Nelson SR, Mackey L, Schlitt JT, Marathe A, Abbas KM, Swarup S. Semantic network analysis of vaccine sentiment in online social media. Vaccine. 2017 Jun 22;35(29):3621-3638. doi: 10.1016/j.vaccine.2017.05.052. Epub 2017 May 27. PMID: 28554500; PMCID: PMC5548132.
Donthu N, Kumar S, Mukherjee D, Pandey N, Lim WM. How to conduct a bibliometric analysis: an overview and guidelines. J Bus Res. 2021;133:285-296.
Joshi S, Nair MK. Prediction of heart disease using classification-based data mining technique. In: Computational Intelligence in Data Mining-Volume 2. Springer; 2015;503-511.
Brachman RJ, Anand T. The process of knowledge discovery in databases: a human-centered approach. In: Advances in Knowledge Discovery and Data Mining. Menlo Park: AAAI Press/The MIT Press; 1996;37-58.
Kokol P, Blažun Vošner H, Završnik J. Application of bibliometrics in medicine: a historical bibliometrics analysis. Health Info Libr J. 2021 Jun;38(2):125-138. doi: 10.1111/hir.12295. Epub 2020 Jan 29. PMID: 31995273.
Garrido-Cardenas JA, de Lamo-Sevilla C, Cabezas-Fernández MT, Manzano-Agugliaro F, Martínez-Lirola M. Global tuberculosis research and its future prospects. Tuberculosis (Edinb). 2020 Mar;121:101917. doi: 10.1016/j.tube.2020.101917. Epub 2020 Feb 23. PMID: 32279873.
Dinic B, Jevremov T. Trends in research related to the dark triad: a bibliometric analysis. Curr Psychol. 2021;40(7):3206-3215.
Mongeon P, Paul-Hus A. The journal coverage of Web of Science and Scopus: a comparative analysis. Scientometrics. 2016;106(1):213-228.
Chunjie L, Jianfeng Z, Lei W, Qiang Y. Cosine normalization: using cosine similarity instead of dot product in neural networks. arXiv. 2017. https://doi.org/10.48550/arXiv.1702.05870.
Zhou X, Zhou M, Huang D, Cui L. A probabilistic model for co-occurrence analysis in bibliometrics. J Biomed Inform. 2022 Apr;128:104047. doi: 10.1016/j.jbi.2022.104047. Epub 2022 Mar 4. PMID: 35257868.
Luukkonen T, Tijssen R, Persson ORT, Sivertsen G. The measurement of international scientific collaboration. Scientometrics. 1993;28(1):15-36.
Kobourov SG. Force-directed drawing algorithms. In: Tamassia R, editor. Handbook of graph drawing and visualization. New York: CRC Press; 2013;383-408.
Yang K, Wang L, Yang G, Jiang X. Research hotspots and trends in nursing education from 2014 to 2020: A co-word analysis based on keywords. J Adv Nurs. 2022 Mar;78(3):787-798. doi: 10.1111/jan.15010. Epub 2021 Sep 12. PMID: 34514616.
de Rezende LB, Blackwell P, Goncalves M. Research focuses, trends, and major findings on project complexity: a bibliometric network analysis of 50 years of project complexity research. Proj Manage J. 2018;49(1):42-49.
Lee Y, Lee Y, Seong G, Stanescu A, Hwang CS. Analysis of GIS research trends in the US with AAG conference presentations from 2000-2019. Geogr J Korea. 2019;53(4):495-508.
Subbarayalu AV, Idhris M, Prabaharan S, Kamalasanan A, Samuel SA, Sakthivel M, Pattukuthu A, Pandiyarajan A, Ali MB, Purushothaman VK, Marimuthu PR. Research trends in the application of artificial intelligence in physical therapy rehabilitation: a bibliometric study. Acta Biomed. 2024;95(2):e2024087.
Nogales A, Rodríguez-Aragón M, García-Tejedor ÁJ. A systematic review of the application of deep learning techniques in the physiotherapeutic therapy of musculoskeletal pathologies. Comput Biol Med. 2024 Apr;172:108082. doi: 10.1016/j.compbiomed.2024.108082. Epub 2024 Jan 29. PMID: 38461697.
Murikipudi M, Azmee AA, Khan MAAH, Pei Y. CBSA: a deep transfer learning framework for assessing post-stroke exercises. In: Proceedings-2024 IEEE/ACM Connected Health: Applications, Systems and Engineering Technologies. Chase; 2024;85-96. doi: 10.1109/CHASE60773.2024.00018.
Lowe SW. The use of artificial intelligence in crafting a novel method for teaching normal human gait. Eur J Physiother. 2024;1-5.
Meng Y, Lee M, Clark HJY, Nguyen C, Kwon S, Schultz S, Pham NS, Schirmer D, Roh EY. Evaluation of range of motion using vision artificial intelligence (AI) in musculoskeletal medicine. J Med Artif Intell. 2024;7:22.
Rossettini G, Rodeghiero L, Corradi F, Cook C, Pillastrini P, Turolla A, Castellini G, Chiappinotto S, Gianola S, Palese A. Comparative accuracy of ChatGPT-4, Microsoft Copilot and Google Gemini in the Italian entrance test for healthcare sciences degrees: a cross-sectional study. BMC Med Educ. 2024 Jun 26;24(1):694. doi: 10.1186/s12909-024-05630-9. PMID: 38926809; PMCID: PMC11210096.
Liang HW, Ameri R, Band S, Chen HS, Ho SY, Zaidan B, Chang KC, Chang A. Fall risk classification with posturographic parameters in community-dwelling older adults: a machine learning and explainable artificial intelligence approach. J Neuroeng Rehabil. 2024 Jan 29;21(1):15. doi: 10.1186/s12984-024-01310-3. PMID: 38287415; PMCID: PMC10826018.
Reis FJJ, Carvalho MBL, Neves GA, Nogueira LC, Meziat-Filho N. Machine learning methods in physical therapy: A scoping review of applications in clinical context. Musculoskelet Sci Pract. 2024 Sep 13;74:103184. doi: 10.1016/j.msksp.2024.103184. Epub ahead of print. PMID: 39278141.
Bekbolatova M, Mayer J, Ong CW, Toma M. Transformative Potential of AI in Healthcare: Definitions, Applications, and Navigating the Ethical Landscape and Public Perspectives. Healthcare (Basel). 2024 Jan 5;12(2):125. doi: 10.3390/healthcare12020125. PMID: 38255014; PMCID: PMC10815906.
Shawli L, Alsobhi M, Faisal Chevidikunnan M, Rosewilliam S, Basuodan R, Khan F. Physical therapists' perceptions and attitudes towards artificial intelligence in healthcare and rehabilitation: A qualitative study. Musculoskelet Sci Pract. 2024 Oct;73:103152. doi: 10.1016/j.msksp.2024.103152. Epub 2024 Jul 24. PMID: 39067366.