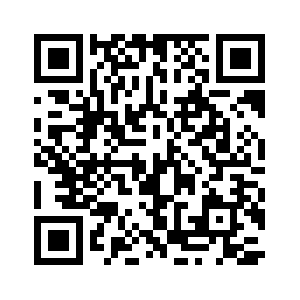
A Study of Multi-Pose Effects On a Face Recognition System
Forensic Medicine受け取った 04 Jul 2024 受け入れられた 24 Jul 2024 オンラインで公開された 25 Jul 2024
ISSN: 2995-8067 | Quick Google Scholar
受け取った 04 Jul 2024 受け入れられた 24 Jul 2024 オンラインで公開された 25 Jul 2024
Interpersonal and intrapersonal face variation interference caused by multiple poses is challenging for distance-based face recognition systems. In this paper, we investigate the face-feature distance distribution for Chinese multi-pose faces. The simulation shows that the number of individuals in the gallery database will greatly affect the recognition performance for near-profile face images. It also provides a prediction of the Top-N occurrence rates in different gallery-size environments.
In single-camera-based face recognition systems, pose variance is the most significant factor affecting the performance of face recognition systems. People look very different from different angles. There are a large number of works on multi-pose face recognition [
, ] use multi-face-recognition classification models on different poses. For example, the left-CNN model [ ] is used to identify the left pose of the human face. For single-model face recognition systems [ , ], face frontalization is the key promising technology to overcome the problem of model degradation owing to the variation of head pose in face recognition. The face frontalization process can be implemented in many different ways [ ] synthesized partial frontal faces and then performed face matching also at the patch level. In [ ], a geometry structure preserving GAN is proposed for multi-pose face frontalization. In [ ], pose face frontalization is performed in feature space. However, most of these methods are stage-wise, which deviates from reality. That makes it difficult to analyze their implications for real-world scenarios. Therefore, we will focus on a simple model FaceNet face recognition system without the face frontalization process. FaceNet system [ ] uses a large dataset of labelled faces to achieve the appropriate pose invariance. In this paper, we will study how pose variation affects the feature distance and recognition performance on a FaceNet-based system. In particular, how it affects the answer to a real-world Top N question in crime scene investigation society. The question is how many candidates need to be investigated in a multi-pose face recognition scenario in order to reach a certain kind of HIT probability.After image acquisition, an individual face image is aligned and cropped to a standard size. Then, the registered face image is transformed into a feature vector. Both the probe face image and all of the gallery face images are transformed in the same way. The distances between the probe image feature and all of the gallery image features are calculated and sorted. The probe image is associated with the N closest gallery candidates for further investigation. It is a very tricky problem to decide the number of N with a certain kind of probability of matching the right person. We will do the statistical simulation test later on and the answer will be in Appendix.
There are many reasons for using n candidates instead of the top one. Time factor: The probe image and the gallery image may not be taken in the same period. Pose factor: Normally, gallery face images are taken under controlled circumstances, with good illumination, front poses, and neutral expressions. However, this is not the case for the probe image. The poses of the probe and gallery faces can be significantly different. A pose change causes a corresponding feature change, which results in distance variation between two feature vectors. The performance of the distance-based face recognition system will inevitably deteriorate.
In this section, the characteristics of pose distance variation will be studied. First, we will look at the intrapersonal multi-pose feature vector distance variation, and next, at the interpersonal variation. The CAS-PEAL Chinese face database [
] is used as the face image data source. The FaceNet model [ ] is used for face feature extraction.Intrapersonal feature vector distance variation: In the PEAL database, there are many possible sources of intrapersonal variation: pure pose, expression, lighting, etc. We study only face images with varying poses, and we measure the vector distance with the front neutral pose. The pose notation we use is the same as in the PEAL paper [
]. PU: the subject is asked to look upward about 30˚; PD: the subject looks downward about 30˚; PM: the subject looks straight ahead. The notation “±nn” indicates the yaw angle of the head.There are approximately 100 individuals in part I of the PEAL database with pose yaw angles of 0°, 22°, 45°, and 67° and approximately 936 individuals in part II with pose yaw angles of 0°, 15°, 30°, and 45°. We perform a simple statistical analysis and show the results in Figure 1 and 2. From these figures, we find that a smaller yaw angle causes a shorter feature distance. The same is true for the pitch angle. A similar result is reported in [
]. Remark: For posing PM00, a few data records do not have zero distance due to the quantization error, and most of them are zero.Interpersonal feature vector distance variation: The feature distances between multi-pose face images and frontal face images from different individuals are calculated. The individuals are chosen at random. Figure 3 shows that the pose variation for the interpersonal distance is very small and negligible.
We compare feature vector distance ranges for the same individual (left side of the grid) and different individuals (right side of the grid) in Figure 4 and find that the distance-based face recognition performance is poor when the interpersonal distance and the intrapersonal distance overlap. Early research [
] also showed that a 45-degree difference in pose between the query image and the database image will make face recognition ineffective.Figure 4 shows how the FaceNet-based system will perform in multi-pose circumstances. If we want to know the detailed implications of the Top N candidates question, we need to perform further calculations. First, we need to know the distance distribution of each pose. Then, we can make Monte Carlo simulations.
Intrapersonal multi-pose distance distribution: We use the MATLAB distribution fitter to plot the probability distribution, which suggests that the data distribution for the intrapersonal multi-pose distance is lognormal. After that, we use the fit function to model different pose distance distributions. The estimated lognormal distribution model parameters are listed in Table 1. The mean (mu) value increases with the yaw angle for all three pitch angles PD, PM, and PU. This makes sense. Face recognition suffers from intra-class imbalances due to the yaw angle and pitch angle pose variations. The influence of the Multi-pose effect caused the bias part of the distance distribution, which is highly correlated with the angle.
Figure 5 shows that the intrapersonal distance data distribution is also seriously influenced by angel differences. For small angel data, the distance distribution nearly overlapped.
Interpersonal multi-pose distance distribution: It is not surprising that we find that the interpersonal distance has a normal distribution. We use the same procedure to estimate the interpersonal distribution and list the model parameters in Table 2. The means (mu) are very similar for different yaw angles and pitch angles.
We have built a FaceNet-based face recognition system called FaceSearch. The FaceSearch system responds to a probe face search request with n (predefined) candidates sorted by short feature vector distances. These are the top N candidates. Usually, investigators need to combine other information for further investigation. It is beneficial to understand the system limitations of multi-pose face recognition and how much the hit probability changes with the value of n.
Many factors affect the recognition performance. Multiple poses may be the major factor, and another factor is the number of individuals in the gallery [
]. When there are more individuals, there are more interpersonal variations to confuse the probe. To evaluate the percentage of correct hits among n candidates, we performed 10k times Monte Carlo simulations for different poses.Figure 6 is the result for 10k Monte Carlo runs on the near-profile view PD67. This intuitive drawing shows that the performance is not very good, even for small galleries (100 individuals). The investigator needs to check the top 35 people to obtain a 90% chance of finding the right one. The first table in the Appendix will give more numerical detail. The situation will be worse when the gallery database becomes larger. In 10000 individual galleries, only a 33.89 percent chance of Hit can be reached within the top 35 people. The result will be much better, and no further engineering is necessary when the pose yaw angle is within 30 degrees. The complete results are listed in the appendix tables of multi-pose face recognition simulation results.
In this paper, we compare interpersonal and intrapersonal multi-pose feature distance variances and conclude that the distance range interference causes a multi-pose performance drop for a distance-based face recognition system. By modeling the multi-pose distance distribution, Monte Carlo simulations for a FaceNet-based system are conducted. The results give the probability of a Hit when the Top-N policy is chosen. The results also show that the number of individuals in the gallery database will severely affect the performance except for the near front face images (e.g., pose PM ± 15). It is still feasible to search multi-pose faces in small galleries without face frontalization.
Although FaceNet is not the latest face recognition algorithm, it is a good starting point to find out the multi-pose system limit for the top N candidate’s policy application. We chose the CAS-PEAL Chinese face database to simulate because our face recognition system is mainly operated in China. Comparing the result with other (e.g. western Caucasian) face databases will be the future work.
Elharrouss O, Almaadeed N, Al-Maadeed S, Khelifi F. Pose-invariant face recognition with multitask cascade networks. Neural Comput Appl. 2022;34(8):6039-6052. doi:10.1007/s00521-022-07668-3.
Guan Y, Fang J, Wu X. Multi-pose face recognition using Cascade Alignment Network and incremental clustering. Signal Image Video Process. 2021;15(1):63-71. doi:10.1007/s11760-020-01832-4.
He H, Liang J, Hou LX, Yunfei L. Multi-pose face reconstruction and Gabor-based dictionary learning for face recognition. Appl Intell. 2023;53(13):16648-16662. doi:10.1007/s10489-023-02954-1.
Tu X, Zhao J, Liu Q, WenjieGuo, GuodongLi, ZhifengLiu, WeiFeng, Jiashi. Joint Face Image Restoration and Frontalization for Recognition. IEEE Trans Circuits Syst Video Technol. 2022;32(3):1285-1298. doi:10.1109/TCSVT.2021.3077655.
Ding C, Xu C, Tao D. Multi-Task Pose-Invariant Face Recognition. IEEE Trans Image Process. 2015;24(3):980-993. doi:10.1109/TIP.2014.2383290.
Luan X, Geng H, Liu L, Li W, Ren M. Geometry Structure Preserving based GAN for Multi-Pose Face Frontalization and Recognition. IEEE Access. 2020;PP(99):1-1. doi:10.1109/ACCESS.2020.2997572.
Zeng K, Wang Z, Han CZ. Implicit space pose consistent transfer network for deep face verification. Pattern Recognit Lett. 2023;176:1-6. doi:10.1016/j.patrec.2022.12.009.
Philbin FSDKJ. FaceNet: A unified embedding for face recognition and clustering. In: 2015 IEEE Conference on Computer Vision and Pattern Recognition (CVPR). Boston, MA, USA; 2015:815-823. doi:10.1109/CVPR.2015.7298682.
Wen G, et al. The CAS-PEAL large-scale Chinese face database and baseline evaluations. IEEE Trans Syst Man Cybern. 2008;38(1):149-161. doi:10.1109/TSMCB.2007.906173.
Limin B, Mingxing J. A Facial Point Detection and Face Recognition Algorithm Based on Multi-pose (in chi). Comput Digit Eng. 2018;46(7):1440-1445,1457. doi:10.3969/j.issn.1672-9722.2018.07.035.
Phillips PJ, Martin A, Wilson CL, Przybocki M. An introduction evaluating biometric systems. Comput. 2000;33(2):56-63. doi:10.1109/2.826988.
Prince SJD, Elder JH, Jonathan W, Felisberti FM. Tied factor analysis for face recognition across large pose differences. IEEE Trans Pattern Anal Mach Intell. 2008;30(6):970-984. doi:10.1109/TPAMI.2007.70753.
Cao Y. A Study of Multi-Pose Effects On a Face Recognition System. IgMin Res. July 25, 2024; 2(7): 667-672. IgMin ID: igmin231; DOI: 10.61927/igmin231; Available at: igmin.link/p231
次のリンクを共有した人は、このコンテンツを読むことができます:
Address Correspondence:
Yichao Cao, Shanghai Research Institute of Criminal Science and Technology, Shanghai Key Laboratory of Crime Scene Evidence, Shanghai, PR China, Email: [email protected]
How to cite this article:
Cao Y. A Study of Multi-Pose Effects On a Face Recognition System. IgMin Res. July 25, 2024; 2(7): 667-672. IgMin ID: igmin231; DOI: 10.61927/igmin231; Available at: igmin.link/p231
Copyright: © 2024 Cao Y. This is an open access article distributed under the Creative Commons Attribution License, which permits unrestricted use, distribution, and reproduction in any medium, provided the original work is properly cited.
Elharrouss O, Almaadeed N, Al-Maadeed S, Khelifi F. Pose-invariant face recognition with multitask cascade networks. Neural Comput Appl. 2022;34(8):6039-6052. doi:10.1007/s00521-022-07668-3.
Guan Y, Fang J, Wu X. Multi-pose face recognition using Cascade Alignment Network and incremental clustering. Signal Image Video Process. 2021;15(1):63-71. doi:10.1007/s11760-020-01832-4.
He H, Liang J, Hou LX, Yunfei L. Multi-pose face reconstruction and Gabor-based dictionary learning for face recognition. Appl Intell. 2023;53(13):16648-16662. doi:10.1007/s10489-023-02954-1.
Tu X, Zhao J, Liu Q, WenjieGuo, GuodongLi, ZhifengLiu, WeiFeng, Jiashi. Joint Face Image Restoration and Frontalization for Recognition. IEEE Trans Circuits Syst Video Technol. 2022;32(3):1285-1298. doi:10.1109/TCSVT.2021.3077655.
Ding C, Xu C, Tao D. Multi-Task Pose-Invariant Face Recognition. IEEE Trans Image Process. 2015;24(3):980-993. doi:10.1109/TIP.2014.2383290.
Luan X, Geng H, Liu L, Li W, Ren M. Geometry Structure Preserving based GAN for Multi-Pose Face Frontalization and Recognition. IEEE Access. 2020;PP(99):1-1. doi:10.1109/ACCESS.2020.2997572.
Zeng K, Wang Z, Han CZ. Implicit space pose consistent transfer network for deep face verification. Pattern Recognit Lett. 2023;176:1-6. doi:10.1016/j.patrec.2022.12.009.
Philbin FSDKJ. FaceNet: A unified embedding for face recognition and clustering. In: 2015 IEEE Conference on Computer Vision and Pattern Recognition (CVPR). Boston, MA, USA; 2015:815-823. doi:10.1109/CVPR.2015.7298682.
Wen G, et al. The CAS-PEAL large-scale Chinese face database and baseline evaluations. IEEE Trans Syst Man Cybern. 2008;38(1):149-161. doi:10.1109/TSMCB.2007.906173.
Limin B, Mingxing J. A Facial Point Detection and Face Recognition Algorithm Based on Multi-pose (in chi). Comput Digit Eng. 2018;46(7):1440-1445,1457. doi:10.3969/j.issn.1672-9722.2018.07.035.
Phillips PJ, Martin A, Wilson CL, Przybocki M. An introduction evaluating biometric systems. Comput. 2000;33(2):56-63. doi:10.1109/2.826988.
Prince SJD, Elder JH, Jonathan W, Felisberti FM. Tied factor analysis for face recognition across large pose differences. IEEE Trans Pattern Anal Mach Intell. 2008;30(6):970-984. doi:10.1109/TPAMI.2007.70753.