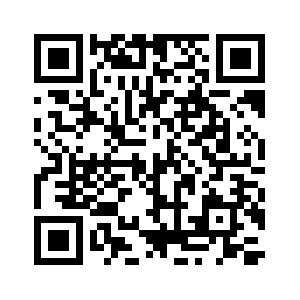
Machine Learning Applied to Electric Vehicle Routing Problem: Optimizing Costs for a Sustainable Environment
Machine Learning Industrial Engineering受け取った 25 Jun 2024 受け入れられた 12 Jul 2024 オンラインで公開された 15 Jul 2024
Focusing on Biology, Medicine and Engineering ISSN: 2995-8067 | Quick Google Scholar
Next Full Text
Levetiracetam-induced Rhabdomyolysis - A Rare Complication
受け取った 25 Jun 2024 受け入れられた 12 Jul 2024 オンラインで公開された 15 Jul 2024
The global move towards Electric Vehicles (EVs) marks a crucial step towards sustainable transportation. However, effectively integrating EVs into the current infrastructure demands more than technological advancements. One of the key challenges is optimizing the routing of EVs to minimize costs and environmental impact. This editorial examines the role of Machine Learning (ML) in addressing the electric vehicle routing problem (ESVRP), highlighting its potential to transform cost optimization and sustainability in transportation. Routing is a fundamental part of transportation logistics, influencing efficiency, cost, and environmental impact. While traditional internal combustion engine vehicles have established routing systems, EVs present unique challenges such as limited battery capacity, longer refueling times, and fewer charging stations. These factors require advanced routing solutions that can dynamically adapt to various constraints.
The EVRP is a variation of the traditional Vehicle Routing Problem (VRP), specifically designed to meet the needs and limitations of electric vehicles. The primary goal of the EVRP is to find the most efficient routes for a fleet of EVs, minimizing costs such as energy consumption, charging time, and operational expenses. Additionally, it aims to enhance sustainability by reducing the environmental footprint of transportation. Machine learning, a subset of artificial intelligence, provides powerful tools to manage the complexities of the EVRP. ML algorithms can process large amounts of data and identify patterns, enabling more accurate predictions and optimal decision-making. Here, we explore how ML can be applied to the EVRP.
A major concern in EV routing is energy consumption. Accurate energy usage predictions are essential for efficient route planning. ML algorithms can analyze historical data, including factors like terrain, traffic conditions, weather, and vehicle load, to predict energy consumption with high precision. These predictions enable route optimization that minimizes energy use and maximizes battery life.
EVs often operate in dynamic environments where conditions can change quickly. Traditional static routing methods are inadequate in such scenarios. ML algorithms, particularly reinforcement learning, can dynamically adjust routes in real-time based on current conditions. For example, if a traffic jam or an unexpected detour occurs, ML can quickly recalculate the most efficient route, ensuring timely deliveries and reducing energy wastage.
The limited availability and varying charging station quality pose significant challenges for EV routing. ML can optimize the use of charging stations by predicting charging demand and recommending the best stations based on factors like location, charging speed, and current availability. Moreover, ML can assist in strategically placing new charging stations where they will be most needed and used.
Efficient scheduling is crucial for businesses managing large fleets of EVs. ML algorithms can optimize fleet management by balancing vehicle availability, maintenance schedules, and charging needs. This comprehensive approach ensures that the fleet operates at maximum efficiency, reducing costs and environmental impact.
Cost optimization in EV routing involves reducing both direct and indirect expenses. Direct costs include energy consumption and vehicle maintenance, while indirect costs encompass factors like time delays and suboptimal charging. ML contributes to cost optimization in several ways:
By accurately predicting energy needs and optimizing routes, ML can significantly reduce energy consumption. This not only lowers operational costs but also extends the lifespan of EV batteries, reducing the frequency of expensive replacements.
Predictive maintenance is another area where ML excels. By analyzing data from vehicle sensors, ML can predict when components are likely to fail and schedule maintenance proactively. This approach minimizes unexpected breakdowns and extends the overall lifespan of the vehicles, reducing maintenance costs.
ML can optimize the allocation of resources, such as drivers and vehicles, ensuring that they are used most efficiently. This reduces idle time, enhances productivity, and cuts down on unnecessary costs.
Sustainability is a core objective of the shift to electric vehicles. ML supports sustainability in EV routing by:
The application of machine learning to the electric vehicle routing problem offers a powerful solution to the dual objectives of cost optimization and environmental sustainability. By leveraging ML’s predictive capabilities, dynamic optimization, and resource management, we can overcome the unique challenges of EV routing. This reduces operational costs and promotes a cleaner and more sustainable transportation system. As we continue to innovate and integrate ML into EV logistics, we move closer to a future where electric vehicles are viable and the preferred transportation choice.
While the application of ML to the EVRP holds great promise, it also presents challenges. Data quality and availability are critical for effective ML models. Ensuring access to accurate and comprehensive data is essential. Moreover, integrating ML solutions with existing transportation systems requires careful planning and collaboration between various stakeholders. Looking forward, the future of ML in EV routing is bright. Advancements in ML algorithms, coupled with increasing data availability from connected vehicles and smart infrastructure, will further enhance the capabilities of ML in addressing the EVRP. Additionally, as renewable energy sources become more prevalent, ML can optimize the use of these sources for charging, further reducing the environmental impact of EVs.
Although AI-generated tools were used to generate this eBook/ Article, the concepts and central ideas it contains were entirely original and devised by a human writer. The AI merely assisted in the writing process, but the creative vision and intellectual property belong to the human author.
Euchi J. Machine Learning Applied to Electric Vehicle Routing Problem: Optimizing Costs for a Sustainable Environment. IgMin Res. July 15, 2024; 2(7): 576-577. IgMin ID: igmin220; DOI: 10.61927/igmin220; Available at: igmin.link/p220
次のリンクを共有した人は、このコンテンツを読むことができます:
Address Correspondence:
Jalel Euchi, OLID Laboratory, Higher Institute of Industrial Management, University of Sfax, Sfax, Tunisia, Email: [email protected]
How to cite this article:
Euchi J. Machine Learning Applied to Electric Vehicle Routing Problem: Optimizing Costs for a Sustainable Environment. IgMin Res. July 15, 2024; 2(7): 576-577. IgMin ID: igmin220; DOI: 10.61927/igmin220; Available at: igmin.link/p220
Copyright: © 2024 Euchi J. This is an open access article distributed under the Creative Commons Attribution License, which permits unrestricted use, distribution, and reproduction in any medium, provided the original work is properly cited.