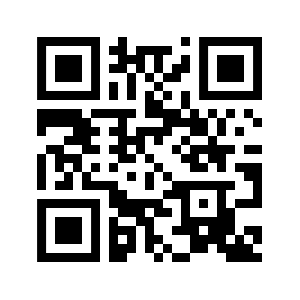
New Scientific Field for Modelling Complex Dynamical Systems: The Cybernetics Artificial Intelligence (CAI)
Artificial Intelligence受け取った 05 Apr 2024 受け入れられた 07 May 2024 オンラインで公開された 08 May 2024
Next Full Text
Roadmap for Greening the Economy of Turkmenistan
受け取った 05 Apr 2024 受け入れられた 07 May 2024 オンラインで公開された 08 May 2024
Artificial Intelligence (AI) has been considered a revolutionary and world-changing science, although it is still a young field and has a long way to go before it can be established as a viable theory. Every day, new knowledge is created at an unthinkable speed, and the Big Data Driven World is already upon us. AI has developed a wide range of theories and software tools that have shown remarkable success in addressing difficult and challenging societal problems. However, the field also faces many challenges and drawbacks that have led some people to view AI with skepticism. One of the main challenges facing AI is the difference between correlation and causation, which plays an important role in AI studies. Additionally, although the term Cybernetics should be a part of AI, it was ignored for many years in AI studies. To address these issues, the Cybernetic Artificial Intelligence (CAI) field has been proposed and analyzed here for the first time. Despite the optimism and enthusiasm surrounding AI, its future may turn out to be a catastrophic Winter for the whole world, depending on who controls its development. The only hope for the survival of the planet lies in the quick development of Cybernetic Artificial Intelligence and the Wise Anthropocentric Revolution. The text proposes specific solutions for achieving these two goals. Furthermore, the importance of differentiating between professional/personal ethics and eternal values is highlighted, and their importance in future AI applications is emphasized for solving challenging societal problems. Ultimately, the future of AI heavily depends on accepting certain ethical values.
Humankind today is confronted with several challenges, threats, risks, and problems that never had faced before [1]. Furthermore, they are global and require cross-institutional solutions. These challenges include issues related to energy, environment, health, ecology, business, economics, international peace, stability, world famine, and spiritual decline just to mention a few. The world is experiencing several catastrophic physical phenomena which increase every year. Humans blame these phenomena on the environment disregarding that it is not the only reason. We share some responsibility for all this. The root causes of all these challenges must be determined carefully, analyzed, and then find sustainable solutions that are valuable and reasonable [1,2].
Today we are often hearing several news with statistics and updates on the problems and challenges of the world. However, in the last 10-15 years there too many issues been added to all these problems. From the recent COVID-19 pandemic and other major health problems to the recent major war in Ukraine and other smaller conflicts in different parts of the world, to climate change, economic crises, high rates of gender inequality, many people living without access to basic needs and medical care, energy shortages, environmental threats, food shortages, and many others. The World Health Organization (WHO), the United Nations (UN), and other world organizations have conducted several studies and have listed numerous serious aspects that the world should be aware of [1]. Which issues are the most important and urgent? Can people solve the problems just by themselves? Certainly not! Working to alleviate global issues does not have to be confusing or stressful. There are various organizations and other established infrastructures to help us see where there are human needs and what resources, and services must be sought and been useful to humanity [2].
Everywhere, there is a feeling of insecurity. Some serious questions are frequently raised such as: how our future life will be? How can we survive on a disturbing and uncertain world? Will new «technologies» leave us without a job? What will happen to our children? [1]. Whether we like it or not, we cannot live the same way as we used to. The life of each person, all structures of the society, the working situations, and the state affairs of governments must be replanned [1,3]. The humankind every day, using already existing well known knowledge evolves and progresses moving forward. In addition, the knowledge been generated everyday by the scientific and academic communities are also at the hands of all citizens of the world to be used for solving their problems and making better their everyday life.
Our main objective is to control and/or exploit natural phenomena or to create human-made objects using new and advanced theories and technologies while at the same time keeping in mind that all our efforts will naturally be useful to humankind. To face and solve all these difficult problems, new theories and methods have emerged during the last 100-120 years. Information Theory (IT) one of the basic concepts of AI was founded by Claude Shannon in 1948 [4]. In his masters thesis at MIT, he demonstrated that the electrical application of Boolean algebra could construct and resolve any logical, numerical device. Cybernetics emerged around the 1950s as a new scientific field [5,6]. Norbert Wiener, considered the father of Cybernetics, named the field after an example of circular causal feedback - that of steering a ship [7]. Cybernetics are embodied in several scientific fields and social issues [8].
Another scientific field was developed at the end of the 1960s and in the 1970s that of Robotics and Mechatronics [9-13]. Robotics is an interdisciplinary branch of computer science and engineering with the main objective to create intelligent machines that can act like humans and assist them in a variety of ways. Since the 1950s first appearance of the term Robotics, the field has evolved and covers a lot of other issues: probabilistic Robotics, [14], the potential of robots for humankind [15], Robots and AI at work [16]. It is of interest to mention studies on holism and chaotic systems: Early work on holism as early as 1926 [17] and the Prigogine theory on chaotic evolution of dynamic systems [18].
In the 1950s, AI was also born [19-22]. Artificial Intelligence (AI) has been considered a revolutionary and world-changing science, although it is still a young field. Most people have had been hoping that all problems of the world will be solved by AI. The truth is that since the 1950s, different scientific fields have emerged to address all the challenges and problems of society. However, there is little interdisciplinary synergy between these different scientific approaches. It is amazing and somehow threatening that AI is behaving as the only player that can confront the problems of the whole world, without taking seriously all other scientific fields. However, the problems of society cannot and have not been carefully analyzed till today. It is a common belief that not a single scientific field can provide valuable solutions. We must wisely and carefully study all problems from a holistic approach, considering all available theories, methods, and techniques. The road is long and full of obstacles. This is the main reason and objective of this overview paper.
The unique features of this paper are:
1) For the first time in the same historical overview paper for artificial intelligence (AI) issues like: Cybernetics, Edge Intelligence, Fuzzy Cognitive Maps, correlation vs. causation, AI Summers and Winters are analyzed and discussed so thoroughly. The findings of this analysis reveal that AI has never till today pay the appropriate attention on the causes creating the available data.
2) For the first time the debate why, Cybernetics is totally ignored by AI is analyzed.
3) The importance of causality in AI is discussed in detail, and it is differentiated from correlation.
4) The use of FCM theories on AI studies are explained.
5) The new term Cybernetics Artificial Intelligence (CAI) is introduced for the first time on an International Journal.
In section 2, a historical review and some ancient myths of AI are presented, while a historical road map for AI is covered in section 3. In section 4 the AI summers and Winters are analyzed also giving a graphical presentation of them over the last 70 years. Section 5 outlines all AI methods and technologies, including for first time the Edge Intelligence (EI) as part of AI. In section 6 the confused meaning of the two extremely important parameters of AI, correlation vs. causation is carefully analyzed for the first time in an overview AI paper. In section 7 the threats of AI are discussed but not extensively since it is not an easy task. In section 8, for the first time in a overview AI paper, the touchy topic of cybernetics been totally ignored by AI architectures and methods is considered and carefully discussed. Section 9 is presenting the new and young scientific field of Fuzzy Cognitive Maps and analyze its usefulness in AI. In section 10 the future of AI is discussed briefly. Finally, section 11 provides some important conclusions and future research directions.
Contrary to popular belief, Artificial Intelligence (AI) was not born just recently. As a matter of fact, Greek mythology is full of myths and stories that refer to the roots of AI [23]. In ancient times master craftsmen were developing intelligent beings according to myths and rumors. Aristotle (384322 B.C.) is considered the father of AI by many people. Aristotle was the first to express accurate set of principles guiding the human mind. Logic is new and necessary reasoning [24,25].
The first humanoid robot in history was the ancient Greek robot, with the name, Talos [23]. Even today, the myth of the bronze giant, Talos, that was also the protector of Minoan Crete is relevant. Talos exemplify the technological achievements in metallurgy during the prehistoric Minoan period. The scientists of that period had reached a high level of technological development and created a bronze superhero to protect them. Talos was not born but was made, either by Zeus himself or Hephaestus, the god of metallurgy and iron, on Zeuss order, according to the myth [23]. In a coin found in the Minoan palace of Phaistos, Talos is portrayed as a young, naked man with wings. Talos body was made of bronze, and he had a single vein that gave him life, starting from his neck and ending in his ankles. Instead of blood, molten metal flowed in his veins, and his ankle had a bronze nail that acted like a stopper to retain this life-giving liquid. Talos primary job was to protect Crete from outside attacks by not allowing ships to approach the island and hurling giant rocks at potential invaders. If an enemy managed to land on the island, Talos body would heat up and glow, and he would kill the invader with a fatal embrace. After killing Cretes enemies, he would break into sarcastic laughter, which was a human characteristic attributed to him. Talos not only protected Crete from outside enemies but also its citizens from all kinds of injustice. Taking power from the wings, Talos would tour Cretan villages three times a year, carrying on his back bronze plates inscribed with divinely inspired laws to ensure their observance in the province. Another important aspect of Talos was that he was serving faithfully justice. This Talos characteristics clearly demonstrates the importance ancient Cretans devoted to justice [23]
Chinese mythology is also present on the history of AI [22,23]. Zhuge Liang , the famous chancellor in Chinese history married a young Chinese girl (Miss. Huang) after been impressed by her cleverness. She was making excellent intelligent machines. When the chancellor went to Huangs house for the first time, he was greeted by two big dogs. The dogs rushed towards him aggressively and were stopped by force by servants of the house. When the chancellor went closer found out that the dogs were actually intelligent machines made of wood. He was impressed by the wisdom of Huang and decided to marry Her. The two intelligent machine dogs present another form of AI in ancient mythical stories. It suggests that humans should have the ability to start or end the operation of artificial intelligent machines. When the two machine dogs received the stop sign by the servants, they stopped immediately. Humans till today still believe that control completely the AI.
Despite all the current hype, AI is not a new scientific field of study. Excluding the purely philosophical reasoning path that extends from Ancient Greek philosophers (such as Aristotle, Plato, Socrates, and others) to Hobbes, Leibniz, and Pascal, AI, as we know AI today. The Dartmouth Summer Research Project on AI, held in the summer of 1956, is universally considered to be the event that founded AI as a scientific field. In Dartmouth, the most preeminent and prestigious experts of that time convened to brainstorm on intelligence theories and simulation. However, to better comprehend and understand AI, it is necessary to investigate theories that predate the 1956 Dartmouth summer workshop. In the late 1940s, there were various names for the field of thinking machines: 1) cybernetics, 2) automata theory, and 3) complex information processing. The variety of names suggests the diversity of conceptual orientations that are paramount in comprehending the issues, challenges, opportunities, risks, and threats of AI. A brief explanation of each will be useful in studying AI.
Cybernetics: The field of Cybernetics has a long history of growth and development, with multiple definitions provided by different experts [26,27]. Cybernetics involves exploring and understanding various systems and their interactions, particularly with regards to circular causality and feedback processes. In such processes, the results of one part of a system serve as input for another part. The term Cybernetics comes from the Greek word for steersman, and was used in ancient texts to signify the governance of people [27]. The French word cybernétique was also used in 1834 to denote the sciences of government in human knowledge classification [28]. The famous Nobert Wiener in 1948, wrote the book Cybernetics, or Control and Communication in the Animal and Machine. In this book the English term Cybernetics was used for the first time [29]. Robert Wiener is considered the father of Cybernetics.
Cybernetics has been used to several fields: engineering, medicine, psychology, international affairs, economics, and architecture. It is often used to comprehend the operation of a process and develop algorithms or models that optimize inputs and minimize delays or overshoots to ensure stability [30]. This understanding of processes is fundamental to optimizing and refining them, making cybernetics a valuable tool in different fields. Margaret Mead emphasized the importance of cybernetics as a cross-disciplinary language, facilitating communication among diverse fields. Understanding and decoding different processes, cybernetics can help refine and optimize them, making it a valuable tool across various fields [31]. Margaret Mead clarified in scientific ways the role of Cybernetics as a form of cross-disciplinary concept. It facilitated easy communication between members of different disciplines, allowing them to speak a common language [32].
Automata theory: The theory of abstract automata, which includes finite automata and other types of automata such as pushdown automata and Turing machines, emerged in the mid-20th century [33]. Automata theory used abstract algebra to describe information systems and their theories unlike previous work on systems theory, which used differential calculus to describe physical systems [34]. The finite-state transducer, which is a type of finite automaton that maps one finite-state machine to another, was developed independently by different research communities under various names [35]. Meanwhile, the concept of Turing machines, which are theoretical models of general-purpose computers, was also included in the discipline of automata theory. Overall, automata theory has had a great influence on computer science and other fields, as it provides a way to formally model and reason about the behavior of computational systems.
Complex information processing: The complex information processing theory is a simplified scientific expression, which compares the human brain to a computer [36]. According to this theory, information processing in the brain occurs in a sequence of stages, like how a computer operates. The sequence has three stages. The first stage involves the receipt of input, which is received by the brain through the senses. The information is then processed in the short-term or working memory, where it can be used to address immediate surroundings or solve problems. In the second stage if the information is deemed important or relevant, it is encoded and stored in the long-term memory for future use. This information can be retrieved and brought back to the working memory when needed using the central control unit. This action can be thought of as the conscious mind. Finally, the output of the system is delivered through an action, like how a computer would deliver an output. While this theory has its limitations and has been criticized by some researchers, it has contributed to our understanding of how information is processed in the brain and has been influential in the development of cognitive psychology and related fields [37,38].
Basics: AI has gone through several peaks and down cycles called AI summers and AI winters respectively. AI from the beginning is running after the dream of building an intelligent machine that can think like, behave like and act like a human. Each AI summer cycle begins with optimistic claims that a fully, generally intelligent machine is just a decade or so away. Funding pours in and progress seems swift. Then, a decade or so later, AI progress stalls and funding dry up. The dream of building such an intelligent machine is postponed for future decades. An AI winter follow and research and funding are reduced [39]. An AI winter ensues, and research and funding are reduced [39]. Figure 1 shows the AI summers and AI winters evolution over the years since the AI conception has been officially accepted by the scientific communities in the summer of 1956 [40].
AI Summers and AI Winters: An AI summer refers to a period when interest and funding for AI experiences a boom, and there are high expectations set for scientific breakthroughs. Most, if not all, significant advancements in AI have occurred during an AI summer. An increase in funding is devoted to the development and application of AI technology. During these periods, great expectations are set due to expected scientific breakthroughs, and pledges are made about the future of AI, leading to market investments [41,42].
The period from 1955 to 1974 is often referred to as the first AI Summer, during which progress appeared to come swiftly as researchers developed computer systems capable of playing chess, deducing mathematical theorems, and even engaging in simple discussions with humans. Government funding flowed generously, and a significant amount of hype was spurred during the mid-50s by a collection of the following AI projects.
1) An AI machine program to translate word-to-word Russian to English languages
2) An AI machine program that could play chess.
3) Crude replications of the human brains neurons by ANNs been consisted of numerous perceptrons.
4) The first humanoid robot, (WABOT), developed by Ichiro Kato of Waseda University, Japan.
During the first AI Summer, the U.S. Defense Advanced Research Projects Agency (DARPA) funded AI research with few requirements for developing functional projects. This AI Summer lasted almost 20 years and saw significant interest and fundamental scientific contributions during what some have called AIs Golden Era. Optimism was so high that in 1970, Minsky famously proclaimed, In three to eight years we will have a machine with the general intelligence of a human being. However, Minskys optimism remained wishful thinking never been met.
Most recent impressive breakthroughs in Artificial Intelligence since roughly 2012, when GPUs and large data sets were first applied, have been based on a specific type of AI algorithm known as Deep Learning (DL). However, even with the fastest hardware configurations and largest data sets available, Machine Learning still faces significant challenges, including sensitivity to adversarial examples and the risk of overfitting or getting stuck in a local minimum. Furthermore, the scientist Geoff Hinton, often called the father of Deep Learning, has raised doubts about the broad applicability of DL and one specific technique used in Convolutional Neural Networks (CNN) called back propagation, stating that he doesnt believe it is how the brain works.
Although AI is currently at the peak of inflated expectations, an AI Winter is likely to follow in the coming years. Its impossible to predict exactly when this winter will occur.
By the mid-1970s, AI progress had stalled as many of the innovations of the previous decade proved too narrow in their applicability, appearing more like toys than steps toward a general version of artificial intelligence. In 1973, the Lighthill Report, an evaluation of academic research in the field of AI, was published [41,42]. It was highly critical of research in the field up to that point, stating that AI research had essentially failed to live up to the grandiose objectives it laid out [42]. Funding dried up so completely that researchers soon stopped using the term AI even on their proposals seeking funding. A book titled Perceptrons: An Introduction to Computational Geometry by Marvin Minsky and Seymour Papert pointed out the flaws and limitations of neural networks. The Lighthill Report and this book influenced DARPA and the UK government to cease funding for AI projects. The first AI Winter had arrived and took place between 1974 and 1980 (Figure 1).
AI winters are periods of time when interest and funding for AI decrease [43]. Several factors are the reason for the arrival of an AI winter, and typically, it is a culmination of factors rather than one sole cause. These factors include hype, overestimation of AI tool capabilities, economic factors, institutional constraints, and a lack of new innovative and creative minds.
Most of the developmental funding during the first AI Summer came from the Defense Advanced Research Projects Agency (DARPA) and the UK government. Early in the 1970s the USA congress forced the stopping of DARPA for AI. Also, in 1973, the Parliament of the United Kingdom asked James Lighthill to review the state of AI in the UK. The Lighthill Report [41] criticized the achievements of AI and proclaimed that all of the worked been performed in the study of AI could be achieved in computer science and in electrical engineering. Additionally, Lighthill reported that the discoveries have not lived up to the hype surrounding them [42]. However, without funding from DARPA and the release of the Lighthill report, the hype surrounding AI could not sustain development, and the world fell into an AI winter. This led to the first notable AI winter in the early 1970s.
Interest in AI would not be revived until years later with the advent of expert systems, which used if-then, rule-based reasoning. An expert system is a program designed to solve problems at a level comparable to that of a human expert in each domain [44,45].
The second AI Summer arrived in the early 1980s, and everything seemed to be heading in the right direction. Funding was increasing, and many scientists were eager to get involved in AI research. New theoretical results were being achieved, and AI was finding its way into many applications across various scientific fields. However, this period lasted less than the first AI Summer and eventually ended with another AI Winter, which lasted from 1987 to 1993, However, in the mid-1990s, AI research began to flourish again. Thus, AI was back on a rising curve, and the summers and winters experienced in the history of AI can be succinctly expressed in the words of Tim Menzies [46]. Since the beginning of the 21st century, most people believe that we have entered a new AI Summer, some even call it the Third AI Summer. The exact starting point of this AI Summer is irrelevant, as we can see from Figure 1 that it began around 2007. We are currently in one of the longest periods of sustained interest in AI in history, with many contributions due to the explosive growth of IT and Industry 4.0, the fourth industrial revolution, and the digitization revolution [47-48].
Most recent impressive breakthroughs in Artificial Intelligence since roughly 2012, when GPUs and large data sets were first applied, have been based on a specific type of AI algorithm known as Deep Learning (DL). However, even with the fastest hardware configurations and largest data sets available, Machine Learning still faces significant challenges, including sensitivity to adversarial examples and the risk of overfitting or getting stuck in a local minimum. Furthermore, the scientist Geoff Hinton, often called the father of Deep Learning, has raised doubts about the broad applicability of DL and one specific technique used in Convolutional Neural Networks (CNN) called back propagation, stating that he doesnt believe it is how the brain works [34,49,50].
While several AI methods and applications will be reviewed in the next section, it remains an open question how far AI technology can go, given these obstacles. Although AI is currently at the peak of inflated expectations, an AI Winter is likely to follow in the coming years. Its impossible to predict exactly when this winter will occur.
The term of AI has never had clear boundaries [21]. Despite that AI has changed over time, the central idea remains the same. One of the main objectives, of AI has always been to build intelligence machines suitable of thinking and acting like humans. AI was introduced at the summer of 1956 in a workshop at Dartmouth College. From that time, it was mainly understood to mean developing an intelligent machine behaving intelligently like a human being. Human beings have demonstrated a unique ability to interpret the physical world around us and use the information we perceive and apprehend to influence changes [22]. Therefore, if we want to develop intelligent machines that can help humans to perform their everyday actions in a better and more efficient way, it is very smart to use humans as a model or prototype.
The growth of AI has been impressive. Attempts to advance AI technologies over the past 50-65 years have emerged in several incredible innovations and developments [51-71]. To comprehend better the several challenging issues of AI, we need to understand well the four basic AI concepts: 1) Machine Learning (ML) 2) Neural Networks (NNs) 3) Deep Learning (DL) and 4) Edge Intelligence (EI). Briefly:
Machine Learning (ML): ML is one of the early and most exciting subfields of AI [72]. ML as subfield of AI entails developing and constructing systems that can learn based on input and output data of the system. Essentially, a ML system learns through observations and experience; actually, according on specific training, the ML system can generalize based on exposure to several cases, and then perform actions in response to new unsought or unforeseen events. AI and ML have been exciting topics for the last 50 years [19-21]. Although both are often used interchangeably [20,21], they are not identical concepts. On one hand AI is a broader concept, that can carry out tasks in a way that can be considered intelligent. On the other hand, ML machine is permitted to have free access to data and using them to be able to learn on their own. ML uses a variety of specific algorithms, frequently organized in taxonomies [72,73].
Neural Networks (NNs): The development of neural networks (NNs) is key to making intelligent machines comprehend the world as humans do. They do this exceptionally well, accurately, fast, and objectively. Historically, NN were motivated by the functionality of the human brain. The first neural network was devised by McCulloch and Pitts [64] to model a biological neuron. Thus, NNs are a set of algorithms modeled loosely after the human brain activities. They interpret sensory data through a kind of machine perception, clustering raw input. A NN can be constructed by linking multiple neurons together in the sense that the output. A simple model for such a network is the multilayer perceptron [53]. NN are constructed to recognize patterns on given data. Over the last few decades, industrial and academic communities have invested a lot of money on NN developments for a wide set of applications obtaining some noticeable results [49,50]. These promising results have been implemented in several fields, such as face recognition, space exploration, optimization, image processing, modeling of nonlinear systems, and also automatic control. More information on NNs can be found in [52,53].
Basics of (DL): It is not an exaggeration to say that some believe that Deep Learning (DL) has revolutionized the world. In other words, AI people think that AI and its methods have been a revolutionary and world-changing science and will provide the world solutions for all problems. The irony is that DL, a surrogate for neural networks (NNs), is an age-old branch of AI that has been resurrected due to several factors such as new and advanced algorithms, fast computer power, and the big data world. Nevertheless, if you want to understand better AI, this DL subfield of AI will help you do so.
DL is one of the most highly sought-after skills in AI technology [49,50,54-57]. The basic idea of DL is simple: the machine learns the features and is usually very good at decision making (classification) versus a human manually designing the system. Today, computer scientists can model many more layers of virtual neurons than ever before thanks to improvements in mathematical algorithms, intelligent theories, and increasingly computer power [58,59].
While AI and ML may appear interchangeable, AI is commonly regarded the universal term, with ML and the other three (3) topics being subsets of AI. Recently Deep Learning (DL) has been considered as a totally new scientific field, which slightly complicates the theoretical foundation of AI concepts [54,55,74]. DL is revisiting AI, ML, and ANNs with the main goal of reformulating all of them due to specific recent scientific and technological developments. DL has been promoted as a buzzword or a rebranding of AI, ML and NNs [75]. Recently, Schmidhuber provides a thorough and extensive overview of DL showing clearly that is a subset of the originally ai definition [60].
Architectures and methods: Today, DL is popular namely for three (3) important reasons: 1) the significantly increased size of data used for training, 2) the drastically increased chip processing abilities (e.g., general-purpose graphical processing units or GPGPUs), and 3) recent developments in ML and signal/information processing research. These three (3) advances have enabled DL methods to effectively exploit complex, compositional nonlinear functions, and make effective use of both structured and unstructured data [61].
There are several DL methods and architectures with the most known:
1) ANNs
2) DNNs
3) CDNNs
4) DBNs
5) RNNs and
6) LSTM
More details and full analysis of the six and other DL methods can be found in [45,48,50-58,72,73,75-81]. However, studying them carefully, all are revised old methods of AI including ML and NN!
A brief historical overview of DL: This historical overview perspective of DL has been chosen to be given now since it will demonstrate the deep roots and strong relationships of DL to AI, ML, NNs, ANNs, and computer vision (CV). The first general, working learning algorithm for supervised deep feedforward multilayer Perceptron was published by Ivakhnenko in 1971 [62]. Other DL working architectures, specifically those built from ANNs, and were introduced by Kunihiko Fukushima in 1980 [63]. Warren McCulloch and Walter Pitts created the first computational model for ANNs based on mathematics and algorithms called threshold logic in 1943 [72]. This was further inspired by the 1959 biological model proposed by Nobel laureates David H. Hubel and Torsten Wiesel [65]. Many ANNs can be considered as cascading models of cell types inspired by certain biological observations [66].
In 1993, Schmidhuber introduced a neural history compressor in the form of an unsupervised stack of Recurrent Neural Networks (RNN), which could solve a Very Deep Learning task requiring more than 1,000 subsequent layers in an RNN unfolded in time [67]. In 1995, Brendan Frey demonstrated that a network containing six fully connected layers and several hundred hidden units could be trained using the wake-sleep algorithm, which was co-developed with Frey, Hinton, and Dayan [68]. However, the training process still took two days.
The real impact of DL in industry apparently began in the early 2000s when CNNs were estimated to have processed 10% to 20% of all checks written in the US in one year. But the industrial application of large-scale speech recognition started around 2010. In late 2009, Li Deng and Geoffrey Hinton got together and worked at Microsoft Research Lab. Along with other researchers of the Microsoft Lab applied DL to speech recognition obtaining some very interesting results. Going one step further, in the same year, they co-organized the 2009 NIPS Workshop on DL for large-scale speech recognition. Two types of systems were found to produce recognition errors with different characteristics, providing scientific understanding on how to integrate DL into the existing highly efficient run-time speech decoding system deployed in the speech recognition industry [69]. Recent books and articles have described and analyzed the history of this significant development in DL, including Deng and Yus article [76]. A comprehensive survey of DL is provided in reference [81]. All these historical remarks demonstrate that DL is not totally a new scientific field; rather, It is part of AI trying to avoid a new AI Winter. Unfortunately, AI will continue to face challenges unless it acknowledges that cybernetics cannot be left out.
Discussions and some criticism on deep learning: Many scientists strongly believe that DL has been marked as a buzzword or a rebranding of NN and AI. Considering the wide-ranging implications of AI, the realization that DL is emerging as one of the most powerful techniques. Thus, DL understandably attracts various discussions, criticisms, and comments. This comes not only from outside the field of computer science but also with insiders from the computer science itself.
A primary criticism of DL regards the mathematical foundations of the algorithms been used. Most methods and algorithms of DL lack of a fundamental theory. Learning in most DL architectures is implemented using gradient descent. While gradient descent has been understood for a while, the theory surrounding other algorithms such as contrastive divergence is less clear (i.e., does it converge? If so, how fast? What is it approximating?). DL methods are often seen as a black box, with most confirmations being done empirically, rather than theoretically.
Other scientists believe that DL should be regarded as a step towards realizing a strong AI, rather than an all-encompassing new method. Gary Marcus a research psychologist remarked that Realistically, DL is only part of the larger challenge of building intelligent machines [74]. The most powerful AI systems, like Watson, use techniques such as DL as just one element of deductive reasoning [69]. It must be noted that all DL algorithms require a large amount of data. However, DL is a useful tool in creating new knowledge [70].
The term edge intelligence, (EI) also known as Edge AI (EAI) and sometimes referred to as intelligence on the edge, is a recent term used in the past few years to describe the merging of machine learning or artificial intelligence with edge computing [71]. Edge Intelligence (EI) is still in its early stages, but the immense possibilities it presents have researchers and companies excited to study and utilize it. The edge has become intelligent through analytics that were once limited to the cloud or in-house data centers. In this process, an intelligent remote sensor node may decide on the spot or send the data to a gateway for further screening before sending it to the cloud or another storage system.
A challenging question is posed here: what are we seeking? Edge Intelligence (EI), Edge Artificial Intelligence (EAI), or simply Intelligence on the Edge? At first glance, this question may cause us to wonder and raise more important questions. Do the three terms refer to the same issues, different issues, or identical ones? How are all these questions and issues related in our pursuit of true scientific knowledge to solve societys problems? Are there any risks associated with scientific knowledge and/or its associated technologies when searching for solutions? And what is their role in the broader scientific area of AI?
We are witnessing an exponential growth of devices, thanks to the advancements in Internet of Things (IoT) technologies, software tools, and hardware architectures. This is having a significant impact on conventional household devices such as smartwatches, smartphones, and smart dishwashers, as well as industrial settings like surveillance cameras, robotic arms, smart warehouses, production conveyor belts, and more. We are now entering an era where devices can independently think, act, and respond in smarter ways, making everything smarter everywhere on the planet.
Before the advent of edge computing, streams of data were sent directly from the Internet of Things (IoT) to a central data storage system. EI or EAI is a new and young subfield of AI and has long way before proves that is useful. Reference [71] conducts a comprehensive survey of recent research efforts on EI or EAI, providing 122 informative references. Reference [82] also discusses EI or EAI and provides 202 references. Thus, these two reference papers indicate that although EI or EAI is in its early stages, it has attracted the interest of many scientists.
The word correlation is commonly used in everyday life to indicate some form of association between variables [83,84]. However, it is astonishing how many people confuse correlation with causation. Journalists are constantly reminded that correlation doesnt imply causation. Yet, these two terms remain one of the most common errors in reporting scientific and health-related studies. In theory, it is easy to distinguish between them - an action or occurrence can cause another (such as smoking causing lung cancer), or it can correlate with another (such as smoking being correlated with high alcohol consumption). If one action causes another, then they are certainly correlated. But just because two things occur together does not mean that one caused the other, even if it seems to make sense. Although correlation does not imply causation, the reverse is always true: causality always implies correlation.
Correlation means association - more precisely it is a measure of the extent to which two variables are related. There are three possible results of a correlational study:
1. Positive correlation If with increase in random variable A, random variable B increases too, or vice versa.
2. Negative correlation If increase in random variable A leads to a decrease in B, or vice versa.
3. Zero or no correlation When both the variables are completely unrelated and change in one lead to no change in other.
Correlation is a statistical measure that shows the relationship between two variables. Pearson coefficient (linear) and Spearman coefficient (non-linear) are two types of correlation coefficients that capture different degrees of probabilistic dependence but not necessarily causation. The correlations coefficients range from -1 (perfect inverse correlation) to 1 (perfect direct correlation), with zero indicating no correlation. You may have observed that the less you sleep, the more tired you are, or that the more you rehearse a skill like dancing, the better you become at it. These simple observations in life form the foundation of correlational research.
Having enough data points and being aware of how the variables interact will determine whether correlations can be used to make predictions. Correlation is the statistical term used to describe the relationship between two quantitative variables. We presume that the relationship is linear and that there is a fixed amount of change in one variable for every unit change in the other. Another method that is frequently employed in these situations is regression, which entails determining the optimal straight line to summarize the association in order to swiftly and effectively rework and rewrite your material [83].
The relationship between random variables A and B can be considered causal if they have a direct cause-and-effect relationship, meaning the existence of one lead to the other. This concept is known as causation or causality. It is crucial for AI to distinguish between correlation and causation because AI methods rely heavily on correlation and large amounts of data. Many AI studies focus on testing correlation but cannot determine a causal relationship. Without considering causation, researchers may arrive at incorrect conclusions regarding the cause of a problem.
Correlation and causation can coexist, as is often the case in our daily lives. However, it is more important to ask what causes the existence of two variables than to establish their correlation. In 2019, Leetaru, K., published an article titled A Reminder That Machine Learning Is About Correlations Not Causation [84], emphasizing the importance of causal AI. To avoid another AI winter that could be catastrophic for humanity, AI needs to integrate cybernetics into its future research studies.
A fundamental question that arises is: How can we establish causality in AI studies? This is one of the most challenging tasks for the academic and scientific communities, and it is crucial for society as a whole, particularly for the manufacturing sector and companies. It requires careful study and analysis by all concerned parties. Some answers can be found in cognitive science, specifically in the emerging scientific field of Fuzzy Logic and Fuzzy Cognitive Maps (FCM) [85,86].
In recent years Artificial Intelligence (AI) has gained much popularity, with the scientific community as well as with the public. Often, AI is ascribed many positive impacts for different social domains such as medicine, energy, and the economy. On the other side, there is also growing concern about its precarious impact on society and individuals, respectively. Several opinion polls frequently query the public fear of autonomous robots and artificial intelligence, a phenomenon coming also into scholarly focus. Indeed, AI has revolutionized industries such as healthcare, finance, manufacturing, and transportation, with its ability to automate and optimize processes, and make predictions and decisions with speed and accuracy.
Despite the fantastic and remarkable AI gains so far, there is still the aguish of what AI could do in the wrong hands [87-92]. One of the biggest concerns with AI is its potential to replace human jobs. As AI systems become more sophisticated, they can perform tasks that were previously thought to require human intelligence, such as analyzing data, making predictions, and even driving vehicles. This could lead to job displacement and unemployment, particularly for workers in low-skilled jobs [88].
Another potential threat of AI is its ability to be used for malicious purposes. For example, AI algorithms can be used to create fake news or deepfake videos, which can be used to spread misinformation and propaganda. AI can also be used to create autonomous weapons, which can operate without human intervention and make decisions about who to target and when to attack.
In addition, there are concerns about the ethics of AI. As AI systems become more complex, they can become difficult to understand and control, which raises questions about who is responsible for their actions. There is also the issue of bias in AI algorithms, which can perpetuate existing inequalities and discrimination.
To address these potential threats, it is important to develop ethical guidelines and regulations for AI. This includes ensuring that AI is developed and used in a way that is transparent, accountable, and respects human rights. It is also important to invest in education and training programs to help workers transition to new industries and roles, as well as to promote digital literacy and critical thinking skills to combat the spread of misinformation. In May 2014, the famed Stephen Hawking, a prominent physicist of the 20th century, gave the world a substantial wakeup shot.
The same year several well-known scientists from all over the planet warned that the intelligent machines been developed for pure commercial use, could be potentially our worst mistake in history. In January 2015, Stephen Hawking, Elon Musk, and several experts of AI signed an open letter calling for research on the impacts of AI in the society [93]. The letter indicated: AI has the potential to eradicate disease and poverty, but researchers must not create something that cannot be controlled [93]. Although we are facing potentially the best or worst thing ever to happen to humanity, and continued: little serious research is devoted to these issues outside small nonprofit institutes. The report had the following important remark: The potential benefits (of AI) are huge since everything that civilization has to offer is a product of human intelligence; we cannot predict what we might achieve when this intelligence is magnified by the tools AI may provide, but the eradication of disease and poverty is not unfathomable. Because of the great potential of AI, it is important to research how to reap its benefits while avoiding potential pitfalls. That was seven (7) years ago! So where are we now? Unfortunately, not much better. The AI threats have not been eliminated but, on the contrary, have increased in numbers and have become more concrete and dangerous [94,95]. This topic is extremely difficult and very sensitive to analyze. There are people who are dead against AI and spread various theories about the AI threats. On the other hand, there are many people who support AI fanatically. Thus, in this paper, only scientists concrete ideas regarding AI threats are stated. In [95], Thomas reports remarks of Elon Musk, founder of Space X, made in a conference, in which he said: Mark my words, AI is far more dangerous than nukes. In the same report [95], several threats of AI are mentioned.
1. Automatic Job losses
2. Violation of private life
3. Algorithmic bias caused by bad data
4. Inequality of social groups
5. Market volatility
6. Development of dangerous Weapons
7. Potential AI Arms Race
8. Stock Market Instability Caused by Algorithmic High Frequency Trading
9. Certain AI methodologies may be fatal to humans
Finally, Stephen Hawking was free and honest in expressing his true feelings and opinions when he told an audience in Portugal that AIs impact could be cataclysmic unless its rapid development is strictly and ethically controlled. Without analysing further, the threats of AI, I would encourage the reader of this paper to seek his own conclusions on all aspects of AI threats and risks. You can start with references [93-102]. See for example references [96-98] and investigate yourself, the recent robot developments, and search for future threats of humanized robots to the society. See also references [87-92].
Overall, while there are potential threats associated with AI, it is important to recognize its potential benefits and to work towards developing responsible and ethical AI systems.
In section 3, three names were given for the field of thinking machines: 1) cybernetics, 2) automata theory, and 3) complex information processing. All three were scientifically developed, analyzed, and defined long before AI as we know it today was officially started in 1956 at Dartmouth College. Of the three names, the one that had been well-known and investigated, at that time, is cybernetics. Artificial Intelligence (AI) is one of the most important and misunderstood sciences of today. Much of this misunderstanding is caused by a failure to recognize its immediate predecessor - Cybernetics. Both AI and Cybernetics are based on binary logic and rely on the same principle for the results they produce: The logical part is universal; the intent is culture specific.
The 1956 Dartmouth Workshop was organized by Marvin Minsky, John McCarthy, and two senior scientists: Claude Shannon and Nathan Rochester of IBM. The proposal for the conference included this assertion: every aspect of learning or any other feature of intelligence can be so precisely described that a machine can be made to simulate it. The conference was attended by 47 scientists, and at the conference, different names for the scientific area were proposed, among them Cybernetics and Logic Theorist. McCarthy persuaded the attendees to accept Artificial Intelligence as the name of the new field. The 1956 Dartmouth conference was the moment that AI gained its name, its mission, its first success, and its major players, and is widely considered the birth of AI. The term Artificial Intelligence was chosen by McCarthy to avoid associations with Cybernetics and connections with the influential cyberneticist Norbert Wiener [29]. McCarthy has said: one of the reasons for inventing the term artificial intelligence was to escape association with cybernetics. Its concentration on analog feedback seemed misguided, and I wished to avoid having either to accept Norbert (not Robert) Wiener as a guru or having to argue with him. Norbert Wiener (November 26, 1894 March 18, 1964) was an American mathematician and philosopher. He was a professor of mathematics at the Massachusetts Institute of Technology (MIT). A child prodigy, Wiener later became an early researcher in stochastic and mathematical noise processes, contributing work relevant to electronic engineering, electronic communication, and control systems. It is astonishing that Wiener was not invited to the 1956 Dartmouth conference.
Although many scientists were aware of the important scientific contributions of Cybernetics, they intentionally chose the term Artificial Intelligence (AI). In recent decades, Cybernetics has often been overshadowed by Artificial Intelligence, even though Artificial Intelligence was influenced by Cybernetics in many ways. Recently, Cybernetics has been returning to the public conscience and is once more being used in multiple fields. It must be emphasized that Cybernetics is an interdisciplinary science that focuses on how a system processes information, responds to it and changes, develops control actions, or restructure the whole system for better functioning. It is a general theory of information processing, feedback control, and decision making. Todays interpretation of the term Cybernetics as it was pioneered by Norbert Wiener in 1948 as the scientific study of control and communication in the animal and the machine is more relevant to our lives today than ever before.
Cybernetics, for many years, has been the science of human-machine interaction that studies and uses the principles of systems control, feedback, identification, and communication. Recently, Cybernetics has been redefined. It has shifted its attention more to the study of regulatory systems that are mechanical, electrical, medical, biological, physical, or cognitive in nature. It studies mainly the concepts of control and communication in living organisms, machines and organizations including self-organization. The question is: Does AI accept the same principles of Cybernetics, and how does it proceed to solve the challenging problems of society? I am afraid to say that although AI is close to Cybernetics, it still fails to provide realistic and viable solutions to the worlds problems. Recently, scientists and mathematicians have begun to think in innovative ways to make machines smarter and approach human intelligence. AI and Cybernetics are perfect examples of this human-machine merger. Binary logic is the main principle in both fields. Both terms are often used interchangeably, but this can cause confusion when studying them. They are slightly different; AI is based on the view that machines can act and behave like humans, while Cybernetics is based on a cognitive view of the world. Further studies on this scientific aspect between AI and Cybernetics will clarify several scientific differences between them.
A more appropriate term for modeling and controlling dynamical complex systems should be Cybernetic Artificial Intelligence (CAI). Such a new scientific field would successfully combine human intelligence (Cybernetics) with machines (AI) in a relatively meaningful and healthy merger. Opening this chapter requires a big effort and innovative ideas.
The most interesting and challenging question of this research study is: why are Fuzzy Cognitive Maps (FCMs) useful in creating new knowledge from the big data-driven world (BDDW) and cyber-physical systems? FCMs possess valuable characteristics that can create new data and knowledge by addressing the cause-and-effect principle, which is the driving force behind most complex dynamic systems. This raises a further question: can FCMs be useful for Artificial Intelligence (AI)? Fuzzy Cognitive Maps are a combination of fuzzy logic and neural networks and were first introduced by Kosko [85] just 35 years ago. It is a very recent scientific technique for simulating complex dynamic systems (CDS), and it exhibits every trait of a CDS and gives a more thorough explanation of FCM in [86]. FCMs are a computational technique that may investigate circumstances when human thought processes contain hazy, fuzzy or ambiguous descriptions. A systems behavior can be simply and symbolically described using an FCM, which gives a graphical depiction of the cause-and-effect relationships between nodes. FCMs, which embody the collective knowledge and expertise of specialists who comprehend how the system operates in various situations, ensure the systems functionality. Language-based variables are used to extract this knowledge, which are subsequently defuzzified into numerical values. In other words, FCMs suggest a modeling approach that consists of a collection of variables (nodes) Ci, as well as the connections (weights) W that connect them. Weights belong in the range [-1, 1], while concepts take values in the range [0, 1]. Figure 2 shows a representative diagram of an FCM. It has been proposed by the author in a number of his publications [3,70,86,103-110].
FCMs may evaluate scenarios in which human thought processes entail fuzzy or uncertain settings using a reasoning process that can manage uncertainty and ambiguity descriptions. FCMs are useful in dealing with complicated dynamic systems.
The full method for the development of a FCM has four (4) steps and is provided in references [86] and [103,111,112].
The sign of each weight Wij represent the type of influence (causality and not correlation) between concepts. There are three types of interconnections between two concepts Ci and Cj and are fully explained in [86].
The absolute value of Wij indicates the degree of influence between two concepts. Ci and Cj. The mathematical formulation calculates the value of each concept using the following equation (1) Refs [86,104,105]:
(1)
where N is the number of concepts, Ai(k + 1) is the value of the concept Ci at the iteration step k+1, Aj(k) is the value of the concept Cj at the iteration step k, Wji is the weight of interconnection from concept Cj to concept Ci and f is the sigmoid function. k1 expresses the influence of the interconnected concepts on the configuration of the new value of the concept Ai and k2 represents the proportion of the contribution of the previous value of the concept in computing the new value. The sigmoid function f is defined with equation (2) Refs. [86,104,105]:
(2)
Where ?>0 determines the steepness of function f. The FCMs concepts are given some initial values which are then changed depending on the weights; the way the concepts affect each other. The calculations stop when a steady state is achieved, the concepts values become stable. A more comprehensive mathematical presentation of FCMs with application to real problems with very useful results is provided in [85,86,103,105-107,111,113-115].
The above methodology and using learning algorithms have been used to create new knowledge, [104,116]. This is the only mathematical model that can describe the dynamic behavior of any system, using recursive equations (eq.1) and the experience of experts with deep knowledge of the system. The experts use methods of cognitive science and fuzzy logic. This approach has been used to address difficult problems with very useful results: in energy [117-119] in health [110,120-128] in business and economics [105,114,129,130], in social and international affairs [81,87-92,106,109,116,131-139], on COVID-19 [107,140-142], in agriculture [108,143,144] in renewable energy sources [145-147]. Reviews of FCMs for several applications are provided in [122,148]. New knowledge is generated not based on statistical analysis and correlation, but on causality and the past knowledge of the system acquired by experts. Neuroscience studies are part of causality and AI methods [83]. Results obtained in several applications using FCM theories and methods with real data, and comparing them with other AI, DL, and ML methods, were 20-25% better. Therefore, FCM theories can be used in combination with AI to merge methods and algorithms to address all societal problems, and thus find viable and realistic solutions. It is important to stress that FCM and AI can create for the first time knew knowledge in a synergistic way [104].
AI has been considered as a world-changing revolution, much like the industrial revolutions that emerged since the early 18th century [149]. We are currently in the 4th IR, or INDUSTRY 4.0, and moving towards INDUSTRY 5.0 or according to some to INDUSTRY 6.0! While all preceding technological revolutions have drastically changed the world, AI is completely different. AI is shaping the future of humanity across nearly every aspect of it, serving as the main driving force for emerging technologies like big data, robotics, nanotechnology, neuroscience, and IoT [150]. AI has gone through summers and winters, and currently, we are in the third (3rd) AI Summer. However, no one can be sure for how long. Some believe we will stay forever in this magnificent AI Summer, while others fear that a new AI Winter is coming, which could be catastrophic for the world.
The question that arises now is what would be the impact of AI on the future of Humanity? Tech giants like Google, Apple, Microsoft, and Amazon are investing annually billions on AI to create several products and services to meet the needs of the society. The state and private sources are pouring generously research funding to many scientists and research institutes. Nevertheless, it is not unclear what the future of AI holds for the planet. Several researchers promise that AI will make the life of the individual better than todays over the next few decades. However, many have serious concerns about how advances in AI will affect what it means to be human, productive, and exercise free will [3,151].
Academicians all over the planet are including AI on their curricula. Researchers are intensifying their efforts in AI and all related subfields of AI, and some developments are already on their way to being fully realized. Still, others are merely theoretical and may remain so. All are disruptive, for better and potentially worse, and theres no downturn in sight. Globalization is at full speed, but it remains to be seen how advances in AI will shape the future of humanity. The digital world is augmenting human capacities and disrupting centuries-old human practices and behaviors. Software-driven systems have spread to more than half of the worlds inhabitants in ambient information and connectivity, offering previously unimagined opportunities but at the same time unprecedented threats.
In the summer of 2018, just five (5) years ago, almost 1000 technology experts from all sectors of society were asked this question, with the year 2030 as the target time. Overall, 63% of respondents said they hope that most individuals will be mostly better off in 2030, while 37% said are afraid that people will not be better off. The experts predicted that advanced AI would amplify human effectiveness but also threaten human freedom, autonomy, agency, and capabilities.
Yes, AI may match or even exceed human intelligence and capabilities in all activities that humans perform today. Indeed, AI smart systems everywhere as well as in many everyday processes will save time, money, and lives. Furthermore, all smart systems will provide opportunities to every individual to enjoy and appreciate a more customized future. But at what expense? No one knows or wants to address these questions from the standpoint of human values and ethics. Todays economic models fail to consider these questions. Most people believe that fundamental inherent values in humans include Love, peace, truth, honesty, loyalty, respect for life, discipline, friendship, and other ethics. These values bring out the fundamental goodness of human beings and society at large [83,109].
Lets face the truth about AI: it can be both constructive and destructive, depending on who is wielding it. We also need to be honest with ourselves. Most AI tools are, and will continue to be, in the hands of companies always aiming for profit or governments seeking to hold political power. Digital systems (also considered smart!) when asked to make decisions for humans and/ or the environment do not take into consideration fundamental ethics and values. As a matter of fact, they have not part of their functionality these ethical issues. Only if the people that own the digital system provide them these values and with instructions how to use them. These smart systems are globally connected and difficult to be controlled or regulated. We need wise leaders who value the human values mentioned above. Questions about privacy, freedom of speech, the right to assemble, and the technological construction of personal life will all re-emerge in this new AI world, especially now that the COVID-19 pandemic is over according to recent statement by the WHO. Who benefits and who is disadvantaged in this new AI world depends on how broadly we analyze these questions today for the future. How can engineers create AI systems that benefit society and are robust? Humans must remain in control of AI; our AI systems must do what we want them to do. The required research is interdisciplinary, drawing from almost all scientific and social areas. This is the reason that in this paper I stress the need to combine the AI and Cybernetics to a new scientific field: the Cybernetics Artificial Intelligence (CAI).
Here are some actions that our society should follow:
1. We should take the open letter from scientists in 2015 seriously [93].
2. Digital cooperation should be prioritized to serve better the needs of the humanity. Human needs must be mathematically formulated and methods to meet these needs must be developed.
3. We need to define the type of society we want to live in.
4. We should strive to develop a new IR, the "Wise Anthropocentric Revolution" [3]. Methods for this new IR are needed.
5. We should develop "smart systems" to avoid divisions between digital "haves" and "have-nots". Software are needed.
6. New economic and political systems are needed to ensure that technology aligns with our values and that AI is in the "right hands." Software tools must be developed.
7. A new scientific field, "Cybernetic Artificial Intelligence" (CAI), should be developed rapidly and vigorously.
8. Edge Intelligence (EI) or Edge AI (EAI) should be developed for each isolated field, such as healthcare, and even for specific topics, such as orthopedics.
9. The new AI world should be governed with people respecting the fundamental huma values and ethics. Mathematical models and methods are needed.
Many more could be developed and pursued by specific groups.
It is up to all of us to work together to develop viable, realistic, reasonable, practical, and wise solutions. We owe it to our children and future generations to do so. To achieve this, we need to accept and follow a solid code of ethics, which sets professionally accepted values, and guiding principles that have been established by superior civilizations for centuries. As the ancient Greek philosopher Plato said: Every science separated from justice and other virtues is cunning, it does not seem to be wisdom. In simpler terms, knowledge without justice and other virtues is better described as cunning than wisdom. This statement expresses the importance of implementing knowledge with justice to turn it into true wisdom.
Progressing in AI without making a similar progress in humanitarian values is like walking with one leg or seeing with just one eye. What an irony! Despite the worlds thirst for peace and sustainable development, people fail to achieve them. But we could if we realize that the solutions to todays problems can be easily found. We only need as free citizens, policy makers, or members of the scientific community, practice philosophy genuinely and satisfactorily addressing and then solve our problems, according to Platos statement. Professional organizations usually establish codes of professional ethics to guide their members in performing their job functions according to sound and consistent ethical principles. The underlying philosophy of having professional ethics is to ensure that individuals in such jobs follow sound, uniform ethical conduct. For example, the Hippocratic Oath taken by medical students is an example of professional ethics that is adhered to even today. Some of the important components of professional ethics that professional organizations typically include in their code of conduct are integrity, honesty, transparency, respectfulness towards the job, fair competition, confidentiality, objectivity, among others. It is important to note that these professional ethics are different from the personal ones mentioned above. In my humble opinion, there is a smiling future for AI if it embraces Cybernetics and the wise anthropocentric ethics and social ethics. In this way I hope we can make AI more human and less artificial.
If we are to discuss future research directions. The most urgent scientific issue is MATERIALS AND METHODS TO BE DEVELOPED for the new proposed CAI. I also propose to start with what Hawking said in 2015 [69]: Comparing the impact of AI on humanity to the arrival of a superior alien species, Hawking and his co-authors found humanitys current state of preparedness deeply wanting. Although we are facing potentially the best or worst thing ever to happen to humanity, they wrote, little serious research is devoted to these issues outside small nonprofit institutes. Furthermore, the future research directions for the AI field are wide open. The proposed nine (9) actions above are only the beginning. Mathematical and scientific approaches from both Cybernetics and AI must be used to realize these fundamental actions. Additionally, for each action, software tools need to be developed and used on real applications. ALL THESE WILL DEVELOPED THE NEEDED MATERIALS AND METHODS OF CAI.
In this historical overview paper on artificial intelligence (AI), a range of issues and concepts have been examined for the first time. Looking back at ancient times, it becomes clear that humanity has always dreamed of creating machines that could mimic living animals and humans. The historical overview provides extensive coverage of all AI developments since the 1956 Dartmouth College conference, which marked the official beginning of AI as we know it today. Although the field of AI could be given many names, many consider AI to be the least accurate of them all. The term Cybernetics has been largely ignored. All of todays methods and algorithms for AI hav have been briefly presented and devoting more scientific material for DL.
Artificial Intelligence (AI) is a relatively young science that follows the traditional historical evolution of any science. Science is built upon theories and models that are formalized with mathematical methods, formulas, and complex equations, formulated by academicians, theoretical scientists, and applied engineers. These theories are then tested and proven useful in society. AI has seen tremendous success in almost all scientific fields, but also faces many challenges, some of which are outlined in this paper. One important challenge is addressing potential failures and striving to prevent them.
In addition, this paper highlights the many open opportunities that lie ahead for AI. AI has experienced its AI Summers and Winters. Today, AI is experiencing an AI Summer. The paper analyzes the two Winters and three AI Summers, and draws lessons from each case. The paper also raises the question of why AI did not take the name Cybernetics and provides a detailed explanation of why the term Cybernetics should be part of AI. The paper proposes a new scientific area combining AI and Cybernetics, called Cybernetic Artificial Intelligence, for the first time.
For the first time, the importance of the young scientific field of Fuzzy Cognitive Maps (FCMs) was presented and briefly formulated. Since FCMs deal with the causal factors that are always present in the dynamic and complex world, it is recommended that AI research should pay serious attention to FCMs. Finally, the future of Artificial Intelligence was considered and briefly analyzed. Despite the optimism and enthusiasm of many people, the future of AI might turn out to be a catastrophic winter for the whole world. It all depends on whose hands AI will be held. The only hope for the survival of the planet is the quick development of Cybernetic Artificial Intelligence and the Wise Anthropocentric Revolution. Some specific solutions for achieving these two goals were given.
Groumpos PE. New Scientific Field for Modelling Complex Dynamical Systems: The Cybernetics Artificial Intelligence (CAI). IgMin Res. May 08, 2024; 2(5): 323-340. IgMin ID: igmin183; DOI:10.61927/igmin183; Available at: igmin.link/p183
次のリンクを共有した人は、このコンテンツを読むことができます:
Emeritus Professor, University of Patras, Greece
Address Correspondence:
Peter P Groumpos, Emeritus Professor, University of Patras, Greece, Email: groumpos@ece.upatras.gr
How to cite this article:
Groumpos PE. New Scientific Field for Modelling Complex Dynamical Systems: The Cybernetics Artificial Intelligence (CAI). IgMin Res. May 08, 2024; 2(5): 323-340. IgMin ID: igmin183; DOI:10.61927/igmin183; Available at: igmin.link/p183
Copyright: © 2024 Groumpos PP. This is an open access article distributed under the Creative Commons Attribution License, which permits unrestricted use, distribution, and reproduction in any medium, provided the original work is properly cited.