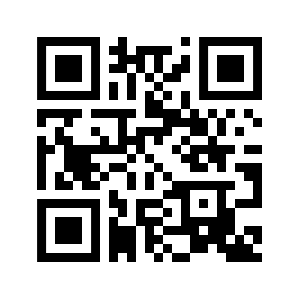
Analytical Expressions of the Markov Chain of K-Ras4B Protein within the Catalytic Environment and a New Markov-State Model
Molecular Biology ProteomicsBiophysics受け取った 11 Dec 2023 受け入れられた 23 Dec 2023 オンラインで公開された 27 Dec 2023
Focusing on Biology, Medicine and Engineering ISSN: 2995-8067 | Quick Google Scholar
受け取った 11 Dec 2023 受け入れられた 23 Dec 2023 オンラインで公開された 27 Dec 2023
The finite Markov chain to which there correspond the qualities of the conformational dynamics of the K-Ras4B proteins in the catalytic reaction is written. The corresponding Markov-Sates models are studied.
The properties of the K-Ras4B processes Markov chain allow one to define a new two-state MSM for the analytical description of the final-state transition. The time evolution of the eigenvalue corresponding to the final-state transition in the Galerkin description is written.
The tools for the analytical calculations of the relative error are therefore prepared.
New analytical formulations of the time evolution of the eigenvalue corresponding to the final-state transition are newly written from the experimental data and form the properties of the lag time in shaping the discretization error. The features of the discretization error are newly studied. A comparison with the experimental data is proposed.
The roles of Ras proteins in transduction pathways and in the subsequent processes are investigated within the framework of oncogenesis in [1].
Further modalities of different K-Ras proteins and environments can be gained insight about from [2], in which the dynamics of the lipids membranes are analyzed within a Hidden-Markov-State Model from [3], and that of biomimetic membranes in [4].
The catalysis properties of the Ras proteins were studied in [5,6]. Ras proteins are used in anticancer drug design [7,8] clinical study for drug discovery is reported to be conducted in [9].
The role of the conformational dynamics in pharmaceutical uses was envisaged in [10].
The catalysis setting examined is one in which the intrinsically-disordered Hyper-viable region (HVR) interacts with the catalytic domain at the effector binding region (as an auto-inhibiting state).
Large-scale conformational transitions of the HVR are studied, from the intrinsically disordered conformation to the ordered one.
The description of the path crucially involves key conformal substrates along the transition pathways; interactions between the HVR and the catalytic domain are considered as being possible.
The states hypothesized as being two possible Markov-States-Model (MSM) states of the K-Ras4B processes were described in [1] after the time-scale test. The description of the disordered part of the dynamical is issued from [11]. Accordingly, the activity at the atomic level gained insight after the analysis of the pathways.
In the present work, the qualities of the time evolution of the K-Ras4B are analytically investigated.
The finite Markov chain corresponding to the K-Ras4B processes is defined after newly spelling out the fundamental matrix and the probability matrix. The MSMs corresponding to the K-Ras4B processes are investigated. A new two-states MSM is here implemented, in order to specify the qualities of the final-state transition, which consists of the M4-M5 MSM: within this new analysis, the time evolution of the eigenvalue in the Galerkin description is spelled out; moreover, new analytical expressions are written after the properties of the MFPT and of the lag time. This way, the new tools to spell the relative error and the discretization error are introduced. A comparison with the experimental data available from [1] is proposed [12]. .
The qualities of the K-RAS proteins are briefly recalled in Section 2. The Markov free-energy-barrier landscape is sketched in Section 3.
The Markov chain originating the Markov states Models is newly found: the fundamental matrix and the probability matrix are newly analytically written in Section 4.
The states of MSMs landscapes are newly analyzed in Section 5; more in detail, the M1-M2-M3-M4-M5 MSM is discussed in Section 6, and the qualities of the M1-M3-M4-M5 MSM are newly spelled out in Section 7.
In Section 8, a new two-states MSM is constructed: the new M4-M5 model is set after the Mean first passage time (MFPT) of the transition in order for the statistical tools to be applied, and the expression of the time evolution of the pertinent eigenvalue in the Galerkin description is spelled.
New expressions of the time evolution of the eigenvalue corresponding to the final-state transition are calculated in Section 9 according to the properties of the MFPT and to those of the lag time, which are newly made use of in the analysis.
The juxtaposition of the perspective studies is presented in Section 11. A comparison with the experimental data is proposed.
The qualities of the K-RAS signal-transducer proteins are recapitulated in [13]; here the dynamics of the K-RAS are reviewed at the atom-lengthscale level and at the conformational-dynamics examination.
The use of the Markv-State Models (MSM) is based on the long time scale statistical dynamics according to the transition probabilities of the protein conformational dynamics explained in [14]: as a result, from [13], 7 metastable states are outlined to be due to sub-states of the switches.
Furthermore, the several K-RAS types of proteins exhibit different behaviors with respect to the metastable states: accordingly, the shift in the K-RAS dynamics is reviewed to happen in an allosteric way.
The differences between the oncogenic K-RAS mutants are juxtaposed in [15-17].
The different binding profiles can be compared within [15,18].
The results interpreted from molecular dynamics simulations might allow one to infer that different mutants have different dynamics [19-21] according to the different Markov landscapes.
The data available from the experiment allow one to make hypotheses about the Markov landscapes of the MSMs fitting the process.
One instance consists of a 4 - state landscape, in which the dynamics take place. More in detail, the states are named M1, M3, M4, M5. The second instance is a 5-state landscape, whose states are named M1, M2, M3, M4, and M5.
The experimental comparison is achieved, according to the investigation of the passage through the state M2.
The Markov chain M originating the process is here newly studied as one composed of 5 states which are analytically written after the partitions of the available portion of the Markov landscape, which are schematised as step functions (on which the path integrals will be calculated).
The first-hitting-time matrix function is spelled from [1].
The fundamental matrix
The fundamental matrix Q of the Markov chain M pertinent to the considered process of transitions is here newly written as
The diagonal entries are controlled to be non-positive.
The probability matrix
The probability matrix P is defined as
(1)
and is here newly written as
In the probability matrix, each row is controlled to be summed as 1.
The processes M1 ? M3 and M2 ? M3 are irreversible; thus, the corresponding entries of the probability matrix are set at p3?1 and p3?2 are set as vanishing; the corresponding modification has to be appointed on the fundamental matrix of the Markov chain as well.
The processes M3 ? M4 and M4 ? M5 are described as irreversible; therefore, the values of the following entries of the probability matrix are set at 1-p34 ? 0 and 1 - p45 = 0.
Because the system is always found to be at the final state M5, then p55=1. The pertinent entries of the matrices are therefore calculated accordingly.
The M1-M2-M3-M4-M5 MSM
The M1-M2-M3-M4-M5 MSM is one containing the five states M1, M2, M3, M4, and M5, which are here newly issued from the Markov chain M. The transition probabilities are those evaluated from the probability matrix P.
The M1-M3-M4-M5 MSM
The Markov chain of this model is here newly set as one for which the transition probabilities pertinent to the state M2 are set to vanish.
The M1-M3-M4-M5 MSM is here newly written as one containing the four states M1, M3, M4, M5 issued from the Markov chain M; this MSM is one obtained after projecting out the vector pertinent to the state M2 out from the Banach space B of M by means of a projector operator; the corresponding Banach space is therefore here found to be one dimension less than that of the M1-M2-M3-M4-M5 scheme.
The new projected probability matrix P is therefore newly written as
Similar to the previous analysis, the transitions M3 ? M4 and M4 ? M5 are experimentally described as irreversible: for this reason, the corresponding probabilities have to be set accordingly.
The new M4-M5 MSM
The transition M4 ? M5 is experimentally validated as irreversible from [1]; it is therefore possible to define the 2 - states MSM constituted of the two states M4 and M5.
The time evolution of the eigenvalue is calculated as the total reward of the path integral (which describes the path followed in the memory-less case). The time evolution of the eigenvalue at the time t is a function of the eigenvalue shift evaluated at the time t + t (and of the time-evolved eigenvalue). The time evolution of the eigenvalue is calculated as the Laplace kernel from [22] and the references therein as one with Radon measure as.
(2)
Where the auxiliary time variable is wanted not to coincide with the exit time (which is implicit for the final state M5): in Eq. (2),
9 New analytical calculations about the time evolution of the final state
It is now possible to calculate the time evolution of the M5 eigenvalue immediately after the time at which the state M5 starts being populated after choosing the lower integration extremum as the time immediately after the (mean) first passage time (MFPT) of the transition M4 ? M5.
This way, the time evolution of the eigenvalue ?5,t is newly obtained after specifying Eq. (2) as
(3)
It is possible to apply here the ingredients from [23], after which it is possible to pose that the time evolution of the eigenvalue at the time t be a function of the eigenvalue shift evaluated at the time t + t only: this way, the time evolution of the eigenvalue ?t is newly obtained after specifying Eq. (2) as
(4)
The description of the Markov landscape as far as the free-energy barriers are concerned was further investigated in [24] and in [25].
The calculation of the path integral (from which the total reward is considered) is here not possible yet, according to the experimental data from [1], from which the qij elements are not spelled out (nor are those of the transition matrix, from which the Q matrix can be extracted after the Kolmogorov equation); nevertheless, the calculations from its reward allow one to pose new further estimates. For the presented purposes, the new two-states MSM is constructed from the states M4 and M5, from which the time evolution of the eigenvalue pertinent to the final-state transition is analytically calculated in the Galerkin description; furthermore, the new estimates are issued from the experimental data available form [1] for the new definitions of the time evolution of the wanted eigenvalues, for which the relative error and the discretization error acquire their physical characterization.
As from [23], the discretization error is expected to increase monotonically with the lag time. As from the accurate estimates from [1], the system was demonstrated to be a Markovian one; in the present work, the detailed specifications of [23] can be in this case also applied, after which the calculation of the discretization error is apt to satisfy the standards requested ibidem. The present work is aimed at analyzing the properties of the dynamics of the K-Ras4B proteins within the framework of the catalytic environment by means of the techniques of the MSM. Different interactions of the proteins, i.e. such as those with the lipids membranes, are described within the framework of a Hidden Markov-State Model. In both cases, a dynamical pattern is individuated among the three first states.
The novelty of the approach here presented is aimed at recovering the items of information about the catalytic mechanism by taking into account the irreversible transition from the penultimate state to the final state. For this purpose, a new two-state MSM was built, which is issued from the Markov chain of K-RAS4B. It is therefore possible to recover the qualities of the eigenvalue of the final transition in the Galerkin representation.
According to this representation, it is possible to retrieve the decay constant and the implied time scale in an analytical manner.
The relative error and the discretization error can be newly analytically calculated according to the qualites of the eigenvalue.
The comparison with the time evolution of the discretization error is therefore newly set.
In [26], the experimental data are scanned, to focus on the inhibition mechanisms of K-RAS(G12D) and its analogs: computer simulations are used to study the conformational space for the cluster analysis by means of a twelve-states MSM. The applications are envisaged to provide guidance for the design of new small molecule inhibitors and to project drugs against K-RAS in general. The analysis of [26,27] demonstrates that there are two clusters within the twelve-state MSM among which the experimental transition probability is high, i.e. one of the transitions C10 ? C12, i.e. the state C11 is considered as not playing a strong role; according to this analysis, it is reasonable to newly postulate a Hidden Markov-State Model which comprehends only the two states, and to further implement the techniques developed of the two-states MSM.
Zhang H, Ni D, Fan J, Li M, Zhang J, Hua C, Nussinov R, Lu S. Markov State Models and Molecular Dynamics Simulations Reveal the Conformational Transition of the Intrinsically Disordered Hypervariable Region of K-Ras4B to the Ordered Conformation. J Chem Inf Model. 2022 Sep 12;62(17):4222-4231. doi: 10.1021/acs.jcim.2c00591. Epub 2022 Aug 22. PMID: 35994329.
López CA, Agarwal A, Van QN, Stephen AG, Gnanakaran S. Unveiling the Dynamics of KRAS4b on Lipid Model Membranes. J Membr Biol. 2021 Apr;254(2):201-216. doi: 10.1007/s00232-021-00176-z. Epub 2021 Apr 7. PMID: 33825026; PMCID: PMC8052243.
Baum LE, Petrie T, Soules G, Weiss N. A Maximization technique occurring in the statistical analysis of probabilistic functions of Markov chains. Ann Math Stat. 1970; 41:164-171.
Shrestha R, Chen D, Frank P, Dwight VN, Thomas JT. Recapitulation of cell-like KRAS4b membrane dynamics on complex biomimetic membranes, iScience. 2022; 25: 1; 103608.
Nussinov R, Zhang M, Maloney R, Jang H. Ras isoform-specific expression, chromatin accessibility, and signaling. Biophys Rev. 2021 Jul 31;13(4):489-505. doi: 10.1007/s12551-021-00817-6. PMID: 34466166; PMCID: PMC8355297.
Chakrabarti M, Jang H, Nussinov R. Comparison of the Conformations of KRAS Isoforms, K-Ras4A and K-Ras4B, Points to Similarities and Significant Differences. J Phys Chem B. 2016 Feb 4;120(4):667-79. doi: 10.1021/acs.jpcb.5b11110. Epub 2016 Jan 27. PMID: 26761128; PMCID: PMC7815164.
Fan J, Liu Y, Kong R, Ni D, Yu Z, Lu S, Zhang J. Harnessing Reversed Allosteric Communication: A Novel Strategy for Allosteric Drug Discovery. J Med Chem. 2021 Dec 23;64(24):17728-17743. doi: 10.1021/acs.jmedchem.1c01695. Epub 2021 Dec 8. PMID: 34878270.
Lu S, Ni D, Wang C, He X, Lin H, Wang Z, Zhang J. Deactivation Pathway of Ras GTPase Underlies Conformational Substates as Targets for Drug Design. ACS Catal. 2019; 9: 7188-7196.
Wang CX, Wang TT, Zhang KD, Li MY, Shen QC, Lu SY, Zhang J. Pan-KRAS inhibitors suppress proliferation through feedback regulation in pancreatic ductal adenocarcinoma. Acta Pharmacol Sin. 2022 Oct;43(10):2696-2708. doi: 10.1038/s41401-022-00897-4. Epub 2022 Mar 29. PMID: 35352018; PMCID: PMC9525295.
Shi Y, Cao S, Ni D, Fan J, Lu S, Xue M. The Role of Conformational Dynamics and Allostery in the Control of Distinct Efficacies of Agonists to the Glucocorticoid Receptor. Front Mol Biosci. 2022 Jul 7;9:933676. doi: 10.3389/fmolb.2022.933676. PMID: 35874618; PMCID: PMC9300934.
Lu S, Banerjee A, Jang H, Zhang J, Gaponenko V, Nussinov R. GTP Binding and Oncogenic Mutations May Attenuate Hypervariable Region (HVR)-Catalytic Domain Interactions in Small GTPase K-Ras4B, Exposing the Effector Binding Site. J Biol Chem. 2015 Nov 27;290(48):28887-900. doi: 10.1074/jbc.M115.664755. Epub 2015 Oct 9. PMID: 26453300; PMCID: PMC4661403.
Ioannis Papastathopoulos, Kirstin Strokorb, Jonathan A. Tawn, Adam Butler, Extreme Events of Markov Chains, Advances in Applied Probability Vol. 49, No. 1 ( 2017), 134-161.
Pantsar T. The current understanding of KRAS protein structure and dynamics. Comput Struct Biotechnol J. 2020; 18: 189-198.
Husic BE, Pande VS. Markov State Models: From an Art to a Science. J Am Chem Soc. 2018 Feb 21;140(7):2386-2396. doi: 10.1021/jacs.7b12191. Epub 2018 Feb 2. PMID: 29323881.
Hunter JC, Manandhar A, Carrasco MA, Gurbani D, Gondi S, Westover KD. Biochemical and Structural Analysis of Common Cancer-Associated KRAS Mutations. Mol Cancer Res. 2015 Sep;13(9):1325-35. doi: 10.1158/1541-7786.MCR-15-0203. Epub 2015 Jun 2. PMID: 26037647.
Winters IP, Chiou SH, Paulk NK, McFarland CD, Lalgudi PV, Ma RK, Lisowski L, Connolly AJ, Petrov DA, Kay MA, Winslow MM. Multiplexed in vivo homology-directed repair and tumor barcoding enables parallel quantification of Kras variant oncogenicity. Nat Commun. 2017 Dec 12;8(1):2053. doi: 10.1038/s41467-017-01519-y. PMID: 29233960; PMCID: PMC5727199.
Haigis KM. KRAS Alleles: The Devil Is in the Detail. Trends Cancer. 2017 Oct;3(10):686-697. doi: 10.1016/j.trecan.2017.08.006. Epub 2017 Sep 12. PMID: 28958387; PMCID: PMC5824632.
Smith MJ, Ikura M. Integrated RAS signaling defined by parallel NMR detection of effectors and regulators. Nat Chem Biol. 2014 Mar;10(3):223-30. doi: 10.1038/nchembio.1435. Epub 2014 Jan 19. PMID: 24441586.
Pantsar T, Rissanen S, Dauch D, Laitinen T, Vattulainen I, Poso A. Assessment of mutation probabilities of KRAS G12 missense mutants and their long-timescale dynamics by atomistic molecular simulations and Markov state modeling. PLoS Comput Biol. 2018 Sep 10;14(9):e1006458. doi: 10.1371/journal.pcbi.1006458. PMID: 30199525; PMCID: PMC6147662.
Prakash P, Gorfe AA. Probing the Conformational and Energy Landscapes of KRAS Membrane Orientation. J Phys Chem B. 2019 Oct 17;123(41):8644-8652. doi: 10.1021/acs.jpcb.9b05796. Epub 2019 Oct 9. PMID: 31554397; PMCID: PMC7045699.
Lu S, Jang H, Nussinov R, Zhang J. The Structural Basis of Oncogenic Mutations G12, G13 and Q61 in Small GTPase K-Ras4B. Sci Rep. 2016 Feb 23;6:21949. doi: 10.1038/srep21949. PMID: 26902995; PMCID: PMC4763299.
Lecian OM. Analytical results from the two-states Markovv-states model and applications to validation of molecular dynamics. International Journal of Mathematics and Computer Research. 2023; 11: 9.
Suárez E, Adelman JL, Zuckerman DM. Accurate Estimation of Protein Folding and Unfolding Times: Beyond Markov State Models. J Chem Theory Comput. 2016 Aug 9;12(8):3473-81. doi: 10.1021/acs.jctc.6b00339. Epub 2016 Jul 11. PMID: 27340835; PMCID: PMC5022777.
Zhang X, Liang W, Zheng G, Li B. Decoding the deactivation mechanism of R192W mutation of ZAP-70 using molecular dynamics simulations and binding free energy calculations. J Mol Model. 2023 Nov 13;29(12):371. doi: 10.1007/s00894-023-05771-6. PMID: 37953318.
Qi X, Li G, Liu J, Mou L, Zhang Y, Guo S, Chen X, Li W. Structural and energetic insights into the selective inhibition of PKMYT1 against WEE1. J Biomol Struct Dyn. 2023 Jun 22:1-9. doi: 10.1080/07391102.2023.2225106. Epub ahead of print. PMID: 37345529.
Liang F, Kang Z, Sun X, Chen J, Duan X, He H, Cheng J. Inhibition mechanism of MRTX1133 on KRASG12D: a molecular dynamics simulation and Markov state model study. J Comput Aided Mol Des. 2023 Mar;37(3):157-166. doi: 10.1007/s10822-023-00498-1. Epub 2023 Feb 28. PMID: 36849761.
Pantsar T, Rissanen S, Dauch D, Laitinen T, Vattulainen I, Poso A. Assessment of mutation probabilities of KRAS G12 missense mutants and their long-timescale dynamics by atomistic molecular simulations and Markov state modeling. PLoS Comput Biol. 2018 Sep 10;14(9):e1006458. doi: 10.1371/journal.pcbi.1006458. PMID: 30199525; PMCID: PMC6147662.
Lecian OR. Analytical Expressions of the Markov Chain of K-Ras4B Protein within the Catalytic Environment and a New Markov-State Model. IgMin Res. 27 Dec, 2023; 1(2): 170-174. IgMin ID: igmin133; DOI: 10.61927/igmin133; Available at: www.igminresearch.com/articles/pdf/igmin133.pdf
次のリンクを共有した人は、このコンテンツを読むことができます:
Address Correspondence:
Orchidea Maria Lecian, Sapienza University of Rome, Rome, Italy, Email: [email protected]
How to cite this article:
Lecian OR. Analytical Expressions of the Markov Chain of K-Ras4B Protein within the Catalytic Environment and a New Markov-State Model. IgMin Res. 27 Dec, 2023; 1(2): 170-174. IgMin ID: igmin133; DOI: 10.61927/igmin133; Available at: www.igminresearch.com/articles/pdf/igmin133.pdf
Copyright: © 2023 Lecian OM. This is an open access article distributed under the Creative Commons Attribution License, which permits unrestricted use, distribution, and reproduction in any medium, provided the original work is properly cited.
Zhang H, Ni D, Fan J, Li M, Zhang J, Hua C, Nussinov R, Lu S. Markov State Models and Molecular Dynamics Simulations Reveal the Conformational Transition of the Intrinsically Disordered Hypervariable Region of K-Ras4B to the Ordered Conformation. J Chem Inf Model. 2022 Sep 12;62(17):4222-4231. doi: 10.1021/acs.jcim.2c00591. Epub 2022 Aug 22. PMID: 35994329.
López CA, Agarwal A, Van QN, Stephen AG, Gnanakaran S. Unveiling the Dynamics of KRAS4b on Lipid Model Membranes. J Membr Biol. 2021 Apr;254(2):201-216. doi: 10.1007/s00232-021-00176-z. Epub 2021 Apr 7. PMID: 33825026; PMCID: PMC8052243.
Baum LE, Petrie T, Soules G, Weiss N. A Maximization technique occurring in the statistical analysis of probabilistic functions of Markov chains. Ann Math Stat. 1970; 41:164-171.
Shrestha R, Chen D, Frank P, Dwight VN, Thomas JT. Recapitulation of cell-like KRAS4b membrane dynamics on complex biomimetic membranes, iScience. 2022; 25: 1; 103608.
Nussinov R, Zhang M, Maloney R, Jang H. Ras isoform-specific expression, chromatin accessibility, and signaling. Biophys Rev. 2021 Jul 31;13(4):489-505. doi: 10.1007/s12551-021-00817-6. PMID: 34466166; PMCID: PMC8355297.
Chakrabarti M, Jang H, Nussinov R. Comparison of the Conformations of KRAS Isoforms, K-Ras4A and K-Ras4B, Points to Similarities and Significant Differences. J Phys Chem B. 2016 Feb 4;120(4):667-79. doi: 10.1021/acs.jpcb.5b11110. Epub 2016 Jan 27. PMID: 26761128; PMCID: PMC7815164.
Fan J, Liu Y, Kong R, Ni D, Yu Z, Lu S, Zhang J. Harnessing Reversed Allosteric Communication: A Novel Strategy for Allosteric Drug Discovery. J Med Chem. 2021 Dec 23;64(24):17728-17743. doi: 10.1021/acs.jmedchem.1c01695. Epub 2021 Dec 8. PMID: 34878270.
Lu S, Ni D, Wang C, He X, Lin H, Wang Z, Zhang J. Deactivation Pathway of Ras GTPase Underlies Conformational Substates as Targets for Drug Design. ACS Catal. 2019; 9: 7188-7196.
Wang CX, Wang TT, Zhang KD, Li MY, Shen QC, Lu SY, Zhang J. Pan-KRAS inhibitors suppress proliferation through feedback regulation in pancreatic ductal adenocarcinoma. Acta Pharmacol Sin. 2022 Oct;43(10):2696-2708. doi: 10.1038/s41401-022-00897-4. Epub 2022 Mar 29. PMID: 35352018; PMCID: PMC9525295.
Shi Y, Cao S, Ni D, Fan J, Lu S, Xue M. The Role of Conformational Dynamics and Allostery in the Control of Distinct Efficacies of Agonists to the Glucocorticoid Receptor. Front Mol Biosci. 2022 Jul 7;9:933676. doi: 10.3389/fmolb.2022.933676. PMID: 35874618; PMCID: PMC9300934.
Lu S, Banerjee A, Jang H, Zhang J, Gaponenko V, Nussinov R. GTP Binding and Oncogenic Mutations May Attenuate Hypervariable Region (HVR)-Catalytic Domain Interactions in Small GTPase K-Ras4B, Exposing the Effector Binding Site. J Biol Chem. 2015 Nov 27;290(48):28887-900. doi: 10.1074/jbc.M115.664755. Epub 2015 Oct 9. PMID: 26453300; PMCID: PMC4661403.
Ioannis Papastathopoulos, Kirstin Strokorb, Jonathan A. Tawn, Adam Butler, Extreme Events of Markov Chains, Advances in Applied Probability Vol. 49, No. 1 ( 2017), 134-161.
Pantsar T. The current understanding of KRAS protein structure and dynamics. Comput Struct Biotechnol J. 2020; 18: 189-198.
Husic BE, Pande VS. Markov State Models: From an Art to a Science. J Am Chem Soc. 2018 Feb 21;140(7):2386-2396. doi: 10.1021/jacs.7b12191. Epub 2018 Feb 2. PMID: 29323881.
Hunter JC, Manandhar A, Carrasco MA, Gurbani D, Gondi S, Westover KD. Biochemical and Structural Analysis of Common Cancer-Associated KRAS Mutations. Mol Cancer Res. 2015 Sep;13(9):1325-35. doi: 10.1158/1541-7786.MCR-15-0203. Epub 2015 Jun 2. PMID: 26037647.
Winters IP, Chiou SH, Paulk NK, McFarland CD, Lalgudi PV, Ma RK, Lisowski L, Connolly AJ, Petrov DA, Kay MA, Winslow MM. Multiplexed in vivo homology-directed repair and tumor barcoding enables parallel quantification of Kras variant oncogenicity. Nat Commun. 2017 Dec 12;8(1):2053. doi: 10.1038/s41467-017-01519-y. PMID: 29233960; PMCID: PMC5727199.
Haigis KM. KRAS Alleles: The Devil Is in the Detail. Trends Cancer. 2017 Oct;3(10):686-697. doi: 10.1016/j.trecan.2017.08.006. Epub 2017 Sep 12. PMID: 28958387; PMCID: PMC5824632.
Smith MJ, Ikura M. Integrated RAS signaling defined by parallel NMR detection of effectors and regulators. Nat Chem Biol. 2014 Mar;10(3):223-30. doi: 10.1038/nchembio.1435. Epub 2014 Jan 19. PMID: 24441586.
Pantsar T, Rissanen S, Dauch D, Laitinen T, Vattulainen I, Poso A. Assessment of mutation probabilities of KRAS G12 missense mutants and their long-timescale dynamics by atomistic molecular simulations and Markov state modeling. PLoS Comput Biol. 2018 Sep 10;14(9):e1006458. doi: 10.1371/journal.pcbi.1006458. PMID: 30199525; PMCID: PMC6147662.
Prakash P, Gorfe AA. Probing the Conformational and Energy Landscapes of KRAS Membrane Orientation. J Phys Chem B. 2019 Oct 17;123(41):8644-8652. doi: 10.1021/acs.jpcb.9b05796. Epub 2019 Oct 9. PMID: 31554397; PMCID: PMC7045699.
Lu S, Jang H, Nussinov R, Zhang J. The Structural Basis of Oncogenic Mutations G12, G13 and Q61 in Small GTPase K-Ras4B. Sci Rep. 2016 Feb 23;6:21949. doi: 10.1038/srep21949. PMID: 26902995; PMCID: PMC4763299.
Lecian OM. Analytical results from the two-states Markovv-states model and applications to validation of molecular dynamics. International Journal of Mathematics and Computer Research. 2023; 11: 9.
Suárez E, Adelman JL, Zuckerman DM. Accurate Estimation of Protein Folding and Unfolding Times: Beyond Markov State Models. J Chem Theory Comput. 2016 Aug 9;12(8):3473-81. doi: 10.1021/acs.jctc.6b00339. Epub 2016 Jul 11. PMID: 27340835; PMCID: PMC5022777.
Zhang X, Liang W, Zheng G, Li B. Decoding the deactivation mechanism of R192W mutation of ZAP-70 using molecular dynamics simulations and binding free energy calculations. J Mol Model. 2023 Nov 13;29(12):371. doi: 10.1007/s00894-023-05771-6. PMID: 37953318.
Qi X, Li G, Liu J, Mou L, Zhang Y, Guo S, Chen X, Li W. Structural and energetic insights into the selective inhibition of PKMYT1 against WEE1. J Biomol Struct Dyn. 2023 Jun 22:1-9. doi: 10.1080/07391102.2023.2225106. Epub ahead of print. PMID: 37345529.
Liang F, Kang Z, Sun X, Chen J, Duan X, He H, Cheng J. Inhibition mechanism of MRTX1133 on KRASG12D: a molecular dynamics simulation and Markov state model study. J Comput Aided Mol Des. 2023 Mar;37(3):157-166. doi: 10.1007/s10822-023-00498-1. Epub 2023 Feb 28. PMID: 36849761.
Pantsar T, Rissanen S, Dauch D, Laitinen T, Vattulainen I, Poso A. Assessment of mutation probabilities of KRAS G12 missense mutants and their long-timescale dynamics by atomistic molecular simulations and Markov state modeling. PLoS Comput Biol. 2018 Sep 10;14(9):e1006458. doi: 10.1371/journal.pcbi.1006458. PMID: 30199525; PMCID: PMC6147662.